Prescreening Questions to Ask AI Bias Specialist
If you're diving into the world of AI and looking to ensure fairness and reduce bias in your models, you've come to the right place. AI bias is a hot topic due to its potential to perpetuate inequality and unfair treatment. To ensure your AI is on the up and up, it's crucial to ask some strong prescreening questions. Let's get started with some key questions you should consider asking any AI expert to gauge their skills and experience in this area.
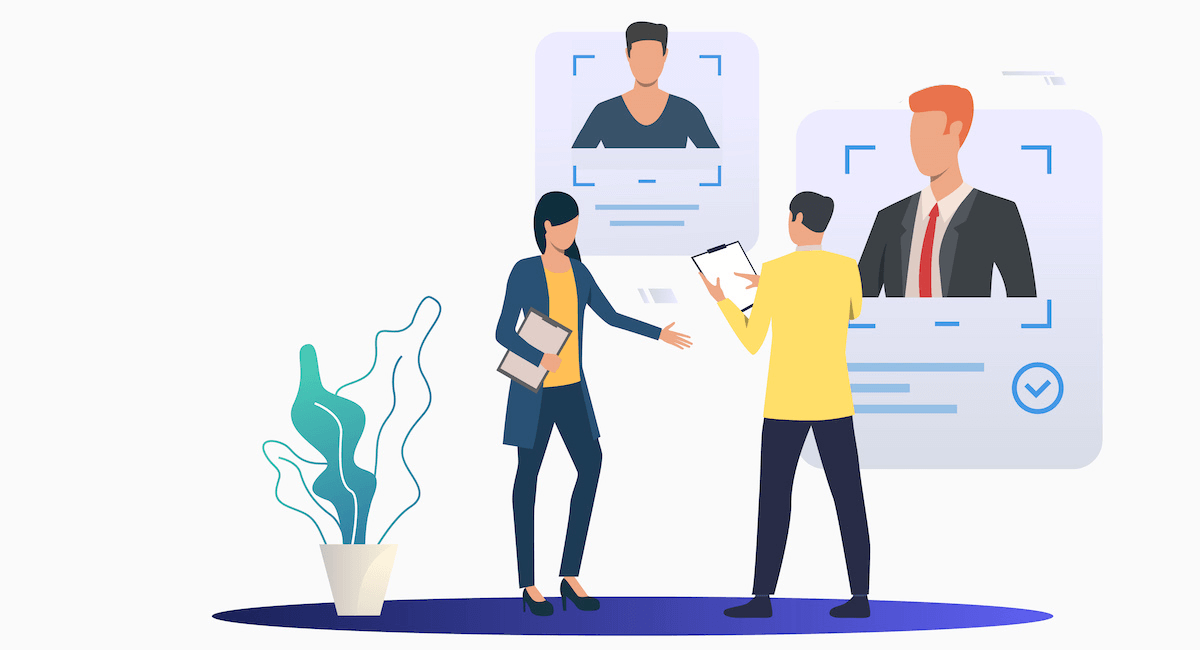
Explain any hands-on experience you have with identifying and mitigating bias in AI models?
First up, let’s talk experience. Ask your candidate to share examples of their past work. Have they tackled bias head-on before? Hearing real-world examples where they've rolled up their sleeves and dug into bias issues can give you confidence in their expertise. Have they used specific techniques or tools that were particularly effective?
What techniques do you utilize to detect bias in datasets prior to model training?
The key to a fair AI model lies in the dataset. Ask about their pre-model training rituals. How do they root out bias in the raw data? Whether it’s through statistical analysis, visualization techniques, or automated tools, understanding their approach can shed light on their thoroughness and methodological soundness.
How familiar are you with fairness metrics in AI, such as disparate impact, demographic parity, or equalized odds?
Fairness metrics are the yardstick for measuring bias. Ask about their familiarity with these metrics. This reveals their depth of knowledge and whether they can apply these concepts to different scenarios. Are they comfortable explaining these metrics and interpreting results?
Tell me about a project where you successfully reduced bias in an AI system. What were your key steps and results?
Practical examples are gold. Encourage the candidate to break down a case study of bias reduction. What specific steps did they take? What were the outcomes? These insights can give you a sense of their strategic approach and effectiveness.
Describe how you would approach auditing an AI model for potential biases.
Ever thought about auditing your AI model like it’s tax season? It’s a necessity! Knowing the candidate’s auditing process helps understand their thoroughness. What checkpoints do they emphasize? How do they ensure nothing slips through the cracks?
How do you ensure the datasets used for training AI are representative of diverse populations?
Diversity is crucial. How does your candidate make sure their datasets are a melting pot of representation? This question delves into their understanding of data collection and preprocessing to include a varied dataset that mirrors real-world diversity.
Can you discuss any tools or frameworks you have used for bias detection and mitigation?
Tools and frameworks are the bread and butter for any AI pro. Ask about the specific ones they’ve used. From IBM’s AI Fairness 360 toolkit to Google's What-If Tool, it's important to know what they have in their arsenal.
How do you stay current with the latest research and developments in AI ethics and bias?
The AI field moves at lightning speed. Look for a candidate who’s plugged into the matrix of latest developments. How do they keep their knowledge fresh? Are they reading research papers, attending conferences, or participating in webinars?
What role does explainability play in mitigating bias within AI systems?
Explainability is like the Rosetta Stone for AI decisions. Ask the candidate how they use explainability to demystify their models. Do they use techniques like LIME or SHAP to make their models’ decisions more transparent? This is vital for trust and bias mitigation.
Share your experience with adversarial training methods and their effectiveness in reducing bias.
Adversarial training is like a boxing match between your AI and bias. What’s their experience in this arena? Understanding their perspective on the effectiveness of adversarial methods can showcase how hands-on they are with advanced techniques.
Describe how you handle scenarios where bias might be introduced during data preprocessing stages.
Data preprocessing is where bias can sneak in like a thief in the night. How do they catch it before it wrecks the model? This question will help you grasp their vigilance during the initial stages of data handling.
Are you comfortable working with multidisciplinary teams, including ethicists, data scientists, and legal experts, to address AI bias?
Bias mitigation isn’t a solo gig. It takes a village. Does the candidate play well with others? Working with ethicists, legal experts, and data scientists is crucial to address AI bias comprehensively.
Can you elaborate on any bias mitigation strategies specific to natural language processing models?
Natural Language Processing (NLP) is a different beast with its unique biases. How does the candidate tackle bias in NLP models specifically? This question helps you assess their specialization and adaptability across AI domains.
Discuss the trade-offs between model accuracy and fairness. How do you navigate these in your work?
Balancing accuracy and fairness is like walking a tightrope. Knowing how the candidate manages this balance can reveal their priorities and decision-making process. Do they have examples where they had to make tough calls between these two crucial aspects?
Have you implemented any bias correction algorithms, such as reweighting or resampling? If so, describe the context and outcomes.
Algorithms for bias correction are part of the toolkit. If the candidate has used methods like reweighting or resampling, get them to spill the details. What was the context, and how effective were these strategies?
When considering bias in AI, how do you balance between different protected attributes (e.g., race, gender, age)?
Different protected attributes require different handling. How does the candidate juggle these variables to ensure balanced bias mitigation? Understanding their approach to multi-dimensional fairness can give you an idea of their comprehensive strategy.
What methods do you recommend for continuous monitoring of AI systems to ensure they remain fair over time?
Bias isn’t a one-time fix. It’s like weeding a garden; it requires ongoing attention. How does the candidate recommend ongoing monitoring? Regular audits, automated checks, or feedback loops – their methods for sustained fairness are crucial.
How do you educate and train other team members or stakeholders about the importance and complexities of AI bias?
Communication is key. How does the candidate demystify AI bias for others? Training and education initiatives indicate their ability to spread awareness and make the intricacies of AI bias accessible to all team members.
Discuss the legal and regulatory implications of AI bias and how they impact your approach to bias mitigation.
The legal landscape around AI is evolving. Ask them about the regulations they're familiar with. How do these laws influence their approach to bias mitigation? Understanding the legal backdrop can provide context to their strategies.
Can you provide an example of a time when you identified an unforeseen bias in an AI system? How did you address it?
No one likes surprises when it comes to bias. Ask them to recount a time they caught an unexpected bias. How did they tackle it? Hearing about their problem-solving skills in action can give you a good sense of their adaptability and expertise.
Prescreening questions for AI Bias Specialist
- Explain any hands-on experience you have with identifying and mitigating bias in AI models.
- What techniques do you utilize to detect bias in datasets prior to model training?
- How familiar are you with fairness metrics in AI, such as disparate impact, demographic parity, or equalized odds?
- Tell me about a project where you successfully reduced bias in an AI system. What were your key steps and results?
- Describe how you would approach auditing an AI model for potential biases.
- How do you ensure the datasets used for training AI are representative of diverse populations?
- Can you discuss any tools or frameworks you have used for bias detection and mitigation?
- How do you stay current with the latest research and developments in AI ethics and bias?
- What role does explainability play in mitigating bias within AI systems?
- Share your experience with adversarial training methods and their effectiveness in reducing bias.
- Describe how you handle scenarios where bias might be introduced during data preprocessing stages.
- Are you comfortable working with multidisciplinary teams, including ethicists, data scientists, and legal experts, to address AI bias?
- Can you elaborate on any bias mitigation strategies specific to natural language processing models?
- Discuss the trade-offs between model accuracy and fairness. How do you navigate these in your work?
- Have you implemented any bias correction algorithms, such as reweighting or resampling? If so, describe the context and outcomes.
- When considering bias in AI, how do you balance between different protected attributes (e.g., race, gender, age)?
- What methods do you recommend for continuous monitoring of AI systems to ensure they remain fair over time?
- How do you educate and train other team members or stakeholders about the importance and complexities of AI bias?
- Discuss the legal and regulatory implications of AI bias and how they impact your approach to bias mitigation.
- Can you provide an example of a time when you identified an unforeseen bias in an AI system? How did you address it?
Interview AI Bias Specialist on Hirevire
Have a list of AI Bias Specialist candidates? Hirevire has got you covered! Schedule interviews with qualified candidates right away.