Prescreening Questions to Ask AI Quality Specialist
So, you're looking to hire an AI quality assurance expert, huh? That's a smart move! Ensuring the quality and reliability of AI systems is no small feat. You need someone who knows their stuff inside and out. Here are some crucial questions to ask your candidates, focusing on their experience, methodologies, and approaches to various challenges. Let's dive in!
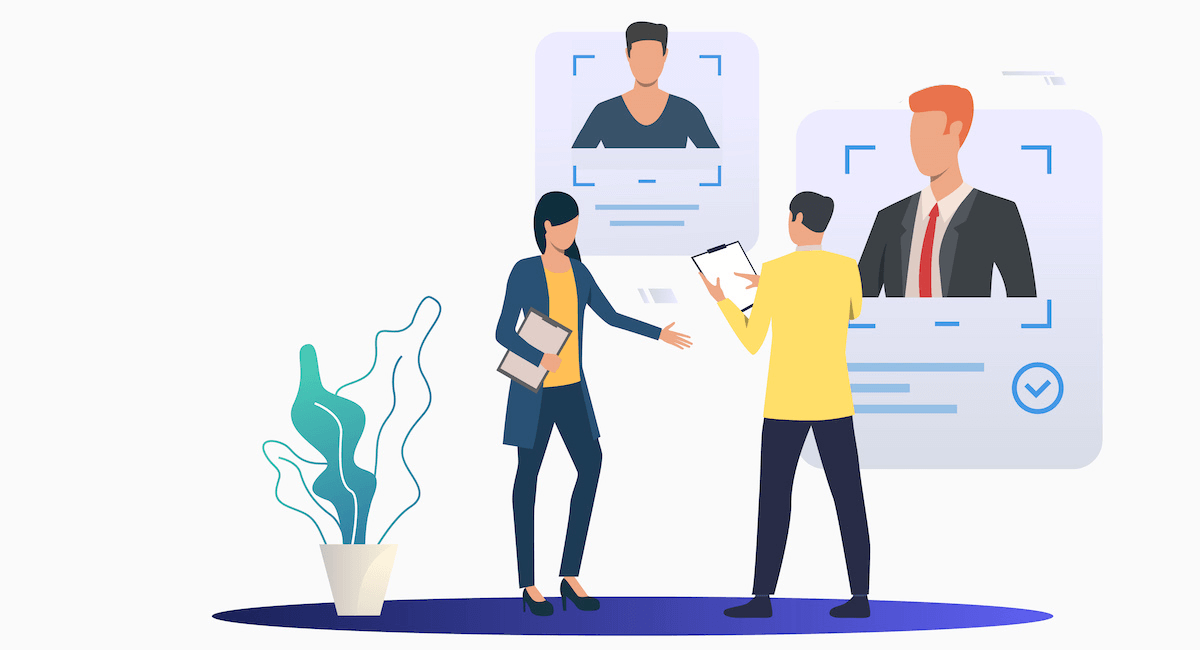
Can you describe your previous experience working with AI systems and ensuring their quality?
This question sets the stage. It's like asking a chef about their favorite recipe; their experience will give you insight into their expertise and practical know-how in handling AI systems. Listen for specifics—they should mention projects, challenges, and the tools they've used.
What methodologies or frameworks do you use to evaluate the performance and reliability of AI models?
Frameworks and methodologies are the backbone of any quality assurance process. Whether it's Six Sigma, Agile, or another framework, their answer will tell you how structured their approach is to ensuring AI efficacy.
How do you approach bias detection and mitigation in AI models?
Bias in AI can be a deal-breaker. Ask this to understand their strategies for identifying and correcting biases, ensuring fairness and ethical standards. It's like asking a detective how they solve crimes—you're looking for a thorough and systematic approach.
Can you provide an example of a challenging AI quality issue you resolved and the steps you took?
Examples speak louder than theories. This question will highlight their problem-solving skills and give you a real-world glimpse into their thought process and capabilities.
How do you stay current with advancements and best practices in AI quality assurance?
AI is a fast-evolving field. Their answer should include reading research papers, attending conferences, participating in webinars, and maybe being part of AI communities. Continuous learning is crucial!
What tools and technologies do you use for monitoring and testing AI systems?
Just as a carpenter relies on tools, an AI specialist needs the right tech to do their job effectively. Look for mentions of specific tools and technologies that help them monitor, test, and ensure AI quality.
How do you ensure that AI models remain accurate and reliable over time?
Maintenance is key. This question touches on their strategies for continuous monitoring, updating models, and ensuring they adapt to new data without losing accuracy.
Can you explain your experience with automating AI quality assurance processes?
Automation can be a game-changer. This will give you insight into their ability to streamline processes, save time, and improve efficiency by implementing automated quality checks.
How do you communicate AI quality metrics and findings to non-technical stakeholders?
Communication is gold. How they explain complex metrics and results in layman's terms is crucial for aligning with business goals and ensuring everyone is on the same page.
Describe your experience with data validation and preprocessing in the context of AI quality.
Good data is the foundation of a good AI model. Their experience in validating and preprocessing data will reveal their diligence and attention to detail.
What key performance indicators (KPIs) do you prioritize when evaluating AI model quality?
KPIs are like a map; they guide you towards your goal. Knowing which metrics they focus on—accuracy, precision, recall, F1 score—will give you an idea of what they consider important in AI quality.
How do you handle discrepancies between expected and actual AI system performance?
Discrepancies are all too common. Their method of handling them—whether it's through debugging, retraining models, or adjusting algorithms—will showcase their resilience and problem-solving skills.
Can you discuss your experience with regulatory compliance in AI development and deployment?
Regulations can be tricky. This question will reveal their familiarity with legal standards and how they ensure compliance, an essential aspect of deploying AI systems responsibly.
What strategies do you use for handling incomplete or noisy datasets?
Data isn't always perfect. Their strategies for managing imperfect data will show their ability to adapt and still deliver reliable AI models despite challenges.
How do you ensure the reproducibility of AI experiments and results?
Reproducibility is critical for validation. Their answer should include methods like version control, detailed documentation, and data logging to ensure results can be consistently replicated.
Can you describe your experience with version control and collaboration tools in AI projects?
Teamwork makes the dream work. Their experience with tools like Git for version control and collaboration platforms like JIRA or Confluence can reveal how well they can work within a team and manage project timelines.
How do you assess the scalability and robustness of AI models?
Scalability and robustness determine how well an AI model performs under variable conditions. Listen for their methods in stress-testing models and ensuring they can handle real-world data pressures.
When encountering an ethical dilemma related to AI development, how do you proceed?
Ethics is non-negotiable. Their approach to handling ethical dilemmas will tell you a lot about their moral compass and their capacity to balance technological possibilities with ethical responsibility.
What is your approach to continuous improvement in AI quality assurance?
Continuous improvement is the hallmark of excellence. Their strategies for learning from past projects, implementing feedback, and constantly enhancing processes will show their commitment to quality.
How do you manage and mitigate risks associated with AI implementations?
Risk is part and parcel of innovation. Their risk management strategies—whether it’s through rigorous testing, multi-stage deployments, or contingency planning—will demonstrate their foresight and preparedness.
Prescreening questions for AI Quality Specialist
- Can you describe your previous experience working with AI systems and ensuring their quality?
- What methodologies or frameworks do you use to evaluate the performance and reliability of AI models?
- How do you approach bias detection and mitigation in AI models?
- Can you provide an example of a challenging AI quality issue you resolved and the steps you took?
- How do you stay current with advancements and best practices in AI quality assurance?
- What tools and technologies do you use for monitoring and testing AI systems?
- How do you ensure that AI models remain accurate and reliable over time?
- Can you explain your experience with automating AI quality assurance processes?
- How do you communicate AI quality metrics and findings to non-technical stakeholders?
- Describe your experience with data validation and preprocessing in the context of AI quality.
- What key performance indicators (KPIs) do you prioritize when evaluating AI model quality?
- How do you handle discrepancies between expected and actual AI system performance?
- Can you discuss your experience with regulatory compliance in AI development and deployment?
- What strategies do you use for handling incomplete or noisy datasets?
- How do you ensure the reproducibility of AI experiments and results?
- Can you describe your experience with version control and collaboration tools in AI projects?
- How do you assess the scalability and robustness of AI models?
- When encountering an ethical dilemma related to AI development, how do you proceed?
- What is your approach to continuous improvement in AI quality assurance?
- How do you manage and mitigate risks associated with AI implementations?
Interview AI Quality Specialist on Hirevire
Have a list of AI Quality Specialist candidates? Hirevire has got you covered! Schedule interviews with qualified candidates right away.