Prescreening Questions to Ask B2B Data Mesh Architect
If you're diving into the world of data mesh architectures or considering a candidate for a position that involves designing and implementing data meshes, you've landed in the right spot. Navigating through the vast ocean of data infrastructure can be challenging, but asking the right questions is like having a compass. Below, we’ll explore some key prescreening questions to ask candidates. Let's make this journey insightful and engaging!
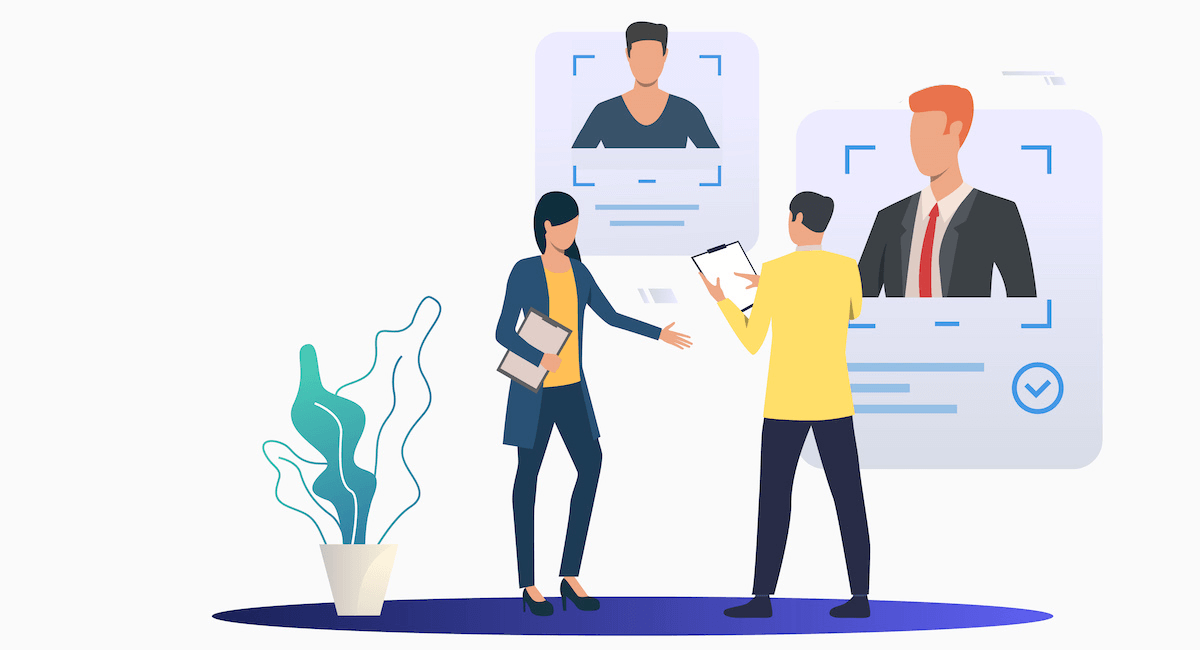
What experience do you have in designing and implementing data mesh architectures?
Diving headfirst into a data mesh initiative without experience is akin to setting sail without a map. This question helps you gauge the candidate's familiarity and hands-on experience with data mesh architecture. Look for responses where candidates can showcase real experiences and highlight specific projects or achievements.
Can you describe a project where you successfully transitioned from a traditional data architecture to a data mesh architecture?
Transitioning from traditional data architectures to a data mesh requires skill, planning, and a whole lot of patience. Here, you want to see if the candidate has navigated this tricky terrain before. Listen for the challenges they faced and their strategies for overcoming them. Maybe they’ve had a few sleepless nights figuring out the kinks – that's what makes them seasoned pros!
How do you ensure data governance and compliance in a data mesh ecosystem?
Data governance is like the captain’s logbook – without it, the crew (data teams) would be lost. Ask candidates how they maintain order, consistency, and compliance within the chaotic yet structured world of data mesh. Their answer will give you insight into their commitment to regulatory standards and data stewardship.
What is your approach to handling data quality and consistency across various data domains?
If data is the new oil, data quality is the refinery. Quality and consistency across domains can make or break a data mesh. Candidates should discuss strategies like data validation, cleaning, and periodic audits to ensure top-notch data across the board.
Can you explain the difference between a data mesh and a data lake or data warehouse?
Ah, the age-old debate! A data mesh isn’t just a fancy buzzword. It’s fundamentally different from data lakes or warehouses. Listen for the candidate’s ability to distinguish these architectures, focusing on decentralized ownership, domain-oriented data, and how data mesh distributes both storage and processing among various teams.
How do you handle data security and privacy in a distributed data architecture?
Of course, with great data comes great responsibility. How a candidate handles security and privacy tells you a lot about their foresight and diligence. They should highlight strategies like encryption, access controls, and monitoring to safeguard data integrity in a data mesh setup.
What tools and technologies have you used for managing data mesh implementations?
Flexing tech muscles, this question will reveal the candidate’s toolbox. Expect to hear about tools like Kafka, Kubernetes, and various cloud services. Their familiarity with tools and tech stacks will hint at their practical knowledge and implementation prowess.
What are the key principles of a data mesh architecture, and how do you implement them?
Understanding the principles of data mesh architecture is like knowing the rules of chess – crucial for winning. Look for answers that cover domain-oriented data ownership, data as a product, self-serve data infrastructure, and federated computational governance. The more detailed, the better.
How do you ensure collaboration and communication between different data teams in a data mesh environment?
Teamwork makes the dream work, right? Communication is key in a data mesh ecosystem. Candidates should talk about fostering a collaborative culture, regular meetings, and the use of collaboration tools to ensure seamless team interactions.
Can you discuss your experience with data cataloging and metadata management in a data mesh?
Data cataloging is like indexing a massive library. Metadata management ensures that data is easily discoverable and usable. Listen for specifics on tools and techniques they’ve used to keep track of data assets in a mesh environment.
What strategies do you use for scaling data mesh architectures as data volume and complexity increase?
Scaling is inevitable as data grows. Candidates need to show how they prepare for that tidal wave. Discuss their tactics for scaling storage, processing power, and community support for a thriving data mesh.
How do you approach integrating legacy systems into a data mesh architecture?
Integrating legacy systems is no walk in the park. It can often feel like fitting a square peg into a round hole. Candidates should describe their strategies for bridging the old with the new, ensuring seamless data flows and minimal disruption.
Can you provide an example of how you've used domain-driven design in a data mesh project?
Domain-driven design is a cornerstone of data mesh. Look for examples where candidates have used domain-oriented thinking to delineate data, streamline processes, and enhance overall efficiency. Their stories will give you a clearer picture of their practical application of these concepts.
What role does data product ownership play in a data mesh, and how do you facilitate it?
Data product ownership ensures accountability and quality. Candidates need to show how they empower data product owners, encourage autonomy, and maintain a balance between central oversight and decentralized execution.
How do you approach monitoring and observability in a data mesh setup?
Think of monitoring and observability as the radar and sonar of your data ship. Candidates should explain their methods for keeping an eye on data flows, detecting anomalies, and ensuring operational health.
What are the biggest challenges you've faced in implementing data mesh, and how did you overcome them?
Every sailor has a stormy tale. Candidates’ anecdotes about challenges will reveal their resilience, creativity, and problem-solving skills. Pay attention to how they navigated obstacles and the lessons they learned along the way.
How do you align data mesh initiatives with business objectives and KPIs?
Speaking the language of business is essential. Candidates should demonstrate how they tie data mesh initiatives with broader business goals, ensuring that their work drives value, meets KPIs, and supports strategic objectives.
Can you discuss your experience with event-driven architectures and their role in data mesh?
Event-driven architectures keep data flowing smoothly, like a well-oiled machine. Candidates should discuss how they’ve utilized event-driven designs to handle real-time data processing and ensure responsiveness in a data mesh.
What is your approach to training and onboarding teams to work effectively with a data mesh?
An effective crew is crucial. Training and onboarding can make or break the adoption of data mesh. Look for candidates who emphasize comprehensive training, hands-on workshops, and continuous support to bring teams up to speed.
How do you balance centralization and decentralization in a data mesh architecture?
Striking the right balance is like walking a tightrope. Candidates need to explain how they manage the tension between centralized policies and the decentralized execution inherent to data mesh. Their approach should ensure harmony and efficiency.
Prescreening questions for B2B Data Mesh Architect
- How do you align data mesh initiatives with business objectives and KPIs?
- What experience do you have in designing and implementing data mesh architectures?
- Can you describe a project where you successfully transitioned from a traditional data architecture to a data mesh architecture?
- How do you ensure data governance and compliance in a data mesh ecosystem?
- What is your approach to handling data quality and consistency across various data domains?
- Can you explain the difference between a data mesh and a data lake or data warehouse?
- How do you handle data security and privacy in a distributed data architecture?
- What tools and technologies have you used for managing data mesh implementations?
- What are the key principles of a data mesh architecture, and how do you implement them?
- How do you ensure collaboration and communication between different data teams in a data mesh environment?
- Can you discuss your experience with data cataloging and metadata management in a data mesh?
- What strategies do you use for scaling data mesh architectures as data volume and complexity increase?
- How do you approach integrating legacy systems into a data mesh architecture?
- Can you provide an example of how you've used domain-driven design in a data mesh project?
- What role does data product ownership play in a data mesh, and how do you facilitate it?
- How do you approach monitoring and observability in a data mesh setup?
- What are the biggest challenges you've faced in implementing data mesh, and how did you overcome them?
- Can you discuss your experience with event-driven architectures and their role in data mesh?
- What is your approach to training and onboarding teams to work effectively with a data mesh?
- How do you balance centralization and decentralization in a data mesh architecture?
Interview B2B Data Mesh Architect on Hirevire
Have a list of B2B Data Mesh Architect candidates? Hirevire has got you covered! Schedule interviews with qualified candidates right away.