Ultimate Guide to Prescreening Questions You Should Ask for Causal AI Scientist
In recent times, the application of Artificial Intelligence has grown exponentially, spreading across various industry verticals. One critical area of AI that has garnered significant attention from data scientists and AI enthusiasts is Causal AI, which refers to the fusion of machine learning with causal inference. This in-depth article aims to address certain prescreening questions potential Causal AI practitioners might have, illuminating central concepts like causal inference, Average Treatment Effect (ATE), Directed Acyclic Graphs (DAGs), 'do-calculus', counterfactual reasoning, and more.
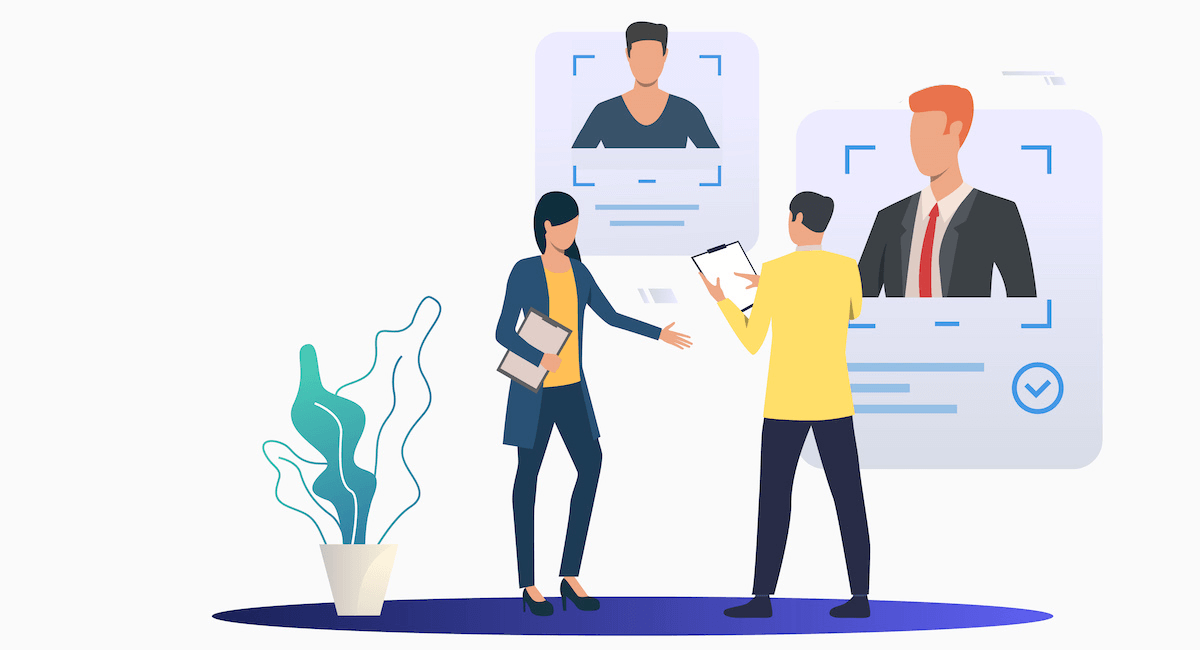
What is your experience with causal inference and machine learning?
Causal inference helps interpret patterns, correlations, and predictions gleaned from machine learning algorithms. While AI models excel at identifying correlations, causal inference takes it a step ahead, explaining why certain occurrences happen, and how these phenomena can be manipulated for improved results.
Can you explain how to estimate Average Treatment Effect (ATE) in a casual AI model?
The Average Treatment Effect (ATE) is a crucial measure in causal AI models. It essentially determines the average difference in outcomes between two groups – one that received treatment, and the other that didn't. It plays a pivotal role in diverse applications, including policy-making, healthcare, and marketing.
Can you give examples of projects where you have used causal AI?
Causal AI has been applied in various domains, such as healthcare, where predictive models help doctors personalize treatments. Similarly, in finance, it aids in risk mitigation and enhancing customer experiences.
What methods have you used to identify causality in a machine learning model?
Techniques like randomized control trials, graphical models, and regression discontinuity are commonly used to deduce causality in machine learning workflows.
How do you handle missing data in causal AI models?
Missing data can skew results and the overall accuracy of machine learning models. Therefore, a range of techniques - including imputation, data deletion, and predictive methods - are employed to handle inconclusive data sets.
What is your understanding of Directed Acyclic Graphs (DAGs) in Causal AI?
DAGs play a crucial role in representing various causal reasoning scenarios in Causal AI. They provide a deterministic and probabilistic graphical model that outlines the cause-effect relationship between different variables.
Could you explain the concept of 'do-calculus' in causality?
'Do-calculus' in Causal AI simply refers to a method that provides a systematic approach to identify and measure the causal effect of certain policies or actions.
Can you describe the difference between exploratory data analysis and causal analysis?
While exploratory data analysis is about describing and summarizing the data, causal analysis delves deeper. It seeks to identify why certain patterns occur in the data, allowing for more refined predictions and strategic decision-making.
Prescreening questions for Causal AI Scientist
- What are the limitations of traditional machine learning when it comes to causality?
- Tell us about an experience when a Causal AI model you implemented drastically enhanced the decision-making process.
- What is your experience with causal inference and machine learning?
- Can you explain how to estimate Average Treatment Effect (ATE) in a casual AI model?
- Can you give examples of projects where you have used causal AI?
- What methods have you used to identify causality in a machine learning model?
- How do you handle missing data in causal AI models?
- What is your understanding of Directed Acycline Graphs (DAGs) in Causal AI?
- Could you explain the concept of 'do-calculus' in causality?
- Can you describe the difference between exploratory data analysis and causal analysis?
- How have you used counterfactual reasoning in your past projects?
- Describe a method to test the validity of causal assumptions
- What techniques have you used for model selection in Causal AI?
- How have you ensured ethical considerations in developing causal AI models?
- Can you describe an instance where you identified and dealt with confounding variables in a causal analysis?
- Can you explain the concept of instrumental variables in determining causality?
- What programming languages are you more comfortable with while working on Causal AI?
- What is your understanding of the backdoor and front-door criteria in determining causal relations?
- Have you ever published or presented any findings relating to Causal AI in a reputable journal or conference?
- What is your understanding of Simpson's paradox in the context of causality analysis?
Interview Causal AI Scientist on Hirevire
Have a list of Causal AI Scientist candidates? Hirevire has got you covered! Schedule interviews with qualified candidates right away.