Prescreening Questions to Ask Data Provenance Analyst
When it comes to data management, there's one word that often pops up: provenance. Whether you're a seasoned data scientist or someone just dipping their toes into the data lake, understanding data provenance and knowing how to handle it are critical skills. Let's dive straight into some essential prescreening questions that you should consider when assessing candidates for your data governance team.
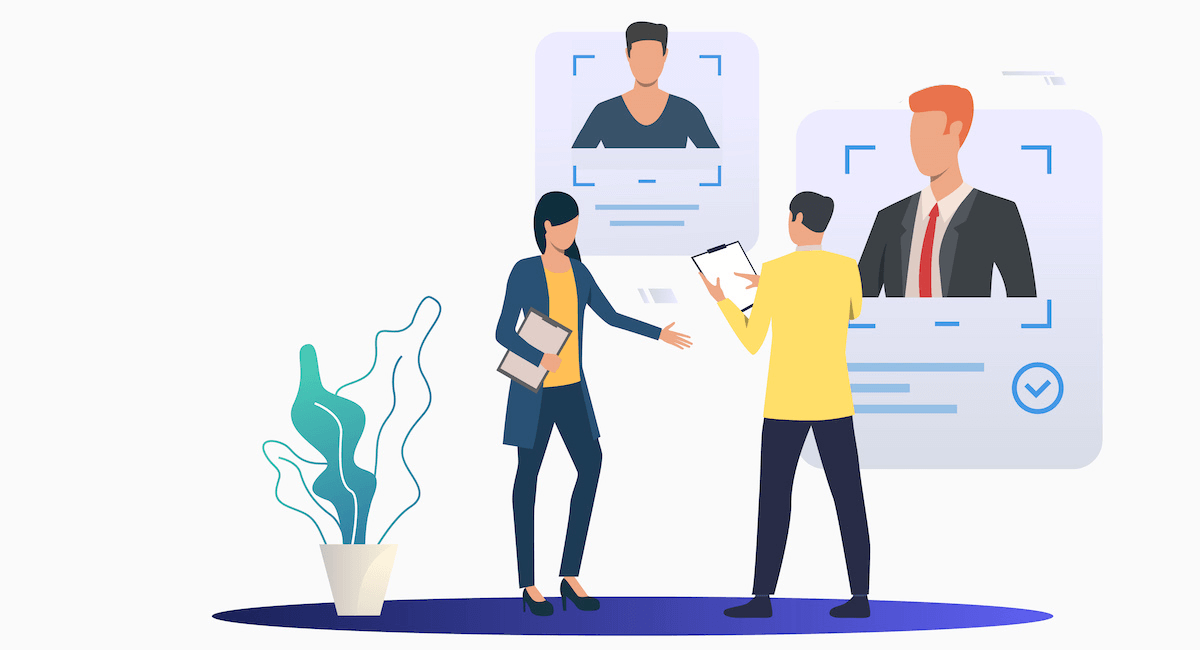
Can you explain the concept of data provenance and its importance in data governance?
Data provenance is essentially the history book of data. It tells you where your data came from, how it has changed over time, and who has interacted with it. Think of it as the "chain of custody" for your data assets. This is crucial because in data governance, knowing the origins and transformations of data helps ensure your data is reliable and trustworthy. Without it, you might as well be driving blindfolded on a foggy night - you're bound to crash!
Describe your experience with tracking data lineage in complex data environments.
Tracking data lineage, especially in complex ecosystems, is like keeping tabs on hundreds of bouncing balls in a chaotic room. It requires meticulous attention to detail and a robust strategy. Share specific examples and instances where you have successfully tracked data lineage, detailing the systems and methods you used to achieve this. Doing so will highlight your hands-on experience and reassure potential employers of your adeptness at handling complexity.
What tools and technologies have you used for data provenance tracking?
There are a host of tools out there designed to make data provenance simpler. From open-source solutions like Apache Atlas to commercial offerings such as Informatica and Alation, each tool has its own set of features and functionalities. Discuss the tools you've had experience with and your proficiency in using them. Your familiarity with these can often be a deal-breaker in landing the job.
How do you ensure the accuracy and integrity of data in your provenance records?
Ensuring data accuracy and integrity is much like guarding a treasure chest. One slip-up and the value diminishes. Talk about the validation checks, reconciliation processes, and audit trails you employ to keep the data honest. Accuracy is not just about catching errors but preventing them from happening in the first place.
Can you describe a challenging data provenance project you worked on and how you approached it?
Everyone loves a good story, especially one with a challenging scenario and a triumphant ending. Share a project where data provenance tracking presented significant hurdles and how you tackled it. This could involve complex data environments, conflicting records, or stringent compliance requirements. Tell your journey from problem to resolution to demonstrate your problem-solving skills and resilience.
What strategies do you use to automate data provenance tracking?
Manual tracking is like using a typewriter when you have a state-of-the-art computer on hand. Automation is key. Discuss the scripts, workflows, and automated tools you use to streamline provenance tracking. Automation not only increases accuracy but also frees up time for more critical tasks, showcasing your efficiency.
How do you handle data provenance in a distributed system or cloud environment?
Distributed systems and cloud environments add layers of complexity to provenance tracking, almost like herding cats. Highlight your strategies for managing this complexity, such as using cloud-native tools and maintaining consistent metadata across distributed systems. Your expertise in this area is invaluable, as more businesses migrate to cloud environments.
What is your experience with metadata management in the context of data provenance?
Metadata is essentially data about data. It's like the nutrition label on your food package. Effective metadata management ensures that every bit of data is correctly classified, tagged, and stored. Discuss your experience in setting up and maintaining robust metadata management systems and how these systems have helped in tracking data provenance.
How do you validate and verify the provenance information collected from various data sources?
Validation and verification are the gatekeepers for data quality. Share your approach to cross-checking and authenticating provenance information from various sources. This could involve automated tools, manual checks, or a combination of both to ensure a high level of data fidelity.
Can you explain the role of data provenance in regulatory compliance (e.g., GDPR, HIPAA)?
Regulatory compliance is a non-negotiable aspect of data governance. Explain how data provenance helps in meeting these requirements by providing an audit trail, thereby ensuring transparency and accountability. Highlight specific regulations like GDPR and HIPAA and how provenance tracking aids in abiding by these laws.
What are the common challenges you face in data provenance and how do you mitigate them?
Challenges in data provenance can range from technological constraints to human errors. Discuss the common hurdles you've encountered, such as data siloing, inconsistent record-keeping, or lack of standardization. More importantly, talk about the measures you've taken to overcome these challenges and ensure smooth tracking.
Describe your experience with data cataloging tools and their role in data provenance.
Data cataloging tools are like your library catalog, making it easy to find what you're looking for. Share the tools you've worked with, such as Collibra or Alation, and how these tools have facilitated better data provenance tracking. Highlighting your experience with these tools underscores your capability to organize and manage data efficiently.
How do you ensure privacy and security while collecting and storing provenance data?
In the age of data breaches and cyber threats, safeguarding provenance data is paramount. Discuss your strategies for ensuring data privacy and security, such as encryption, access controls, and compliance with data protection regulations. Proving that you can protect sensitive information will add a feather to your cap.
Can you explain the difference between data provenance and data lineage?
Data provenance and data lineage are often used interchangeably, but they aren't the same. Think of data lineage as a map showing where the data has been and the transformations it has undergone, while data provenance is the detailed story behind that map, including the who, what, and why. Clarify the distinctions to showcase your deep understanding of both concepts.
What best practices do you follow for documenting data provenance?
Documentation is like a lighthouse guiding the ship. Without it, everything goes astray. Share the best practices you follow, such as maintaining detailed records, using standardized templates, and ensuring regular updates. Good documentation practices are essential for effective data governance and smooth audits.
How do you handle provenance data for unstructured or semi-structured data sources?
Unstructured and semi-structured data can be tricky, like trying to herd wild animals. Discuss your methods for tracking provenance in these formats, whether through specialized tools or unique tagging systems. Your ability to handle diverse data types will demonstrate your versatility and technical prowess.
Describe how you collaborate with other teams (e.g., data engineers, data scientists) on provenance matters.
Teamwork makes the dream work. Share how you collaborate with other roles, such as data engineers and data scientists, to ensure accurate and comprehensive provenance tracking. Whether through regular meetings, collaborative tools, or joint workflows, highlight your ability to work well in a team environment.
What are the key attributes you capture to maintain thorough data provenance records?
Capturing the right attributes is like having the right ingredients for a recipe. Discuss the key attributes you capture, such as data source, timestamp, user ID, and transformation details. Thorough record-keeping ensures you can trace the data journey accurately, mitigating risks and enhancing data quality.
How do you prioritize which datasets or processes require detailed provenance tracking?
Not all data is equal. Prioritization is critical. Explain your approach to determining which datasets or processes need detailed provenance tracking. Factors could include regulatory requirements, business impact, or data sensitivity. Your ability to prioritize shows you can manage resources wisely while ensuring compliance and data integrity.
Can you provide an example of how data provenance helped resolve a data quality issue?
Real-world examples resonate the most. Discuss a specific instance where data provenance helped you identify and resolve a data quality issue. Perhaps it was an inconsistency in historical data or unauthorized data manipulation. Showcasing how provenance tracking led to a solution demonstrates its practical value and your expertise in data governance.
Prescreening questions for Data Provenance Analyst
- Can you explain the concept of data provenance and its importance in data governance?
- Describe your experience with tracking data lineage in complex data environments.
- What tools and technologies have you used for data provenance tracking?
- How do you ensure the accuracy and integrity of data in your provenance records?
- Can you describe a challenging data provenance project you worked on and how you approached it?
- What strategies do you use to automate data provenance tracking?
- How do you handle data provenance in a distributed system or cloud environment?
- What is your experience with metadata management in the context of data provenance?
- How do you validate and verify the provenance information collected from various data sources?
- Can you explain the role of data provenance in regulatory compliance (e.g., GDPR, HIPAA)?
- What are the common challenges you face in data provenance and how do you mitigate them?
- Describe your experience with data cataloging tools and their role in data provenance.
- How do you ensure privacy and security while collecting and storing provenance data?
- Can you explain the difference between data provenance and data lineage?
- What best practices do you follow for documenting data provenance?
- How do you handle provenance data for unstructured or semi-structured data sources?
- Describe how you collaborate with other teams (e.g., data engineers, data scientists) on provenance matters.
- What are the key attributes you capture to maintain thorough data provenance records?
- How do you prioritize which datasets or processes require detailed provenance tracking?
- Can you provide an example of how data provenance helped resolve a data quality issue?
Interview Data Provenance Analyst on Hirevire
Have a list of Data Provenance Analyst candidates? Hirevire has got you covered! Schedule interviews with qualified candidates right away.