Key Prescreening Questions to Ask Genomic Data Scientist for Optimum Candidate Selection
Genomics, the analysis of the structures, functions, and interrelationships of genomes, is a complex field that requires advanced analytical skills and a solid grasp on biological concepts. As such, hiring the right people for your genomic data analyst positions is essential. To help you get started, we've compiled a list of prescreening questions that you can use to assess your candidates' technical competencies and practical experiences.
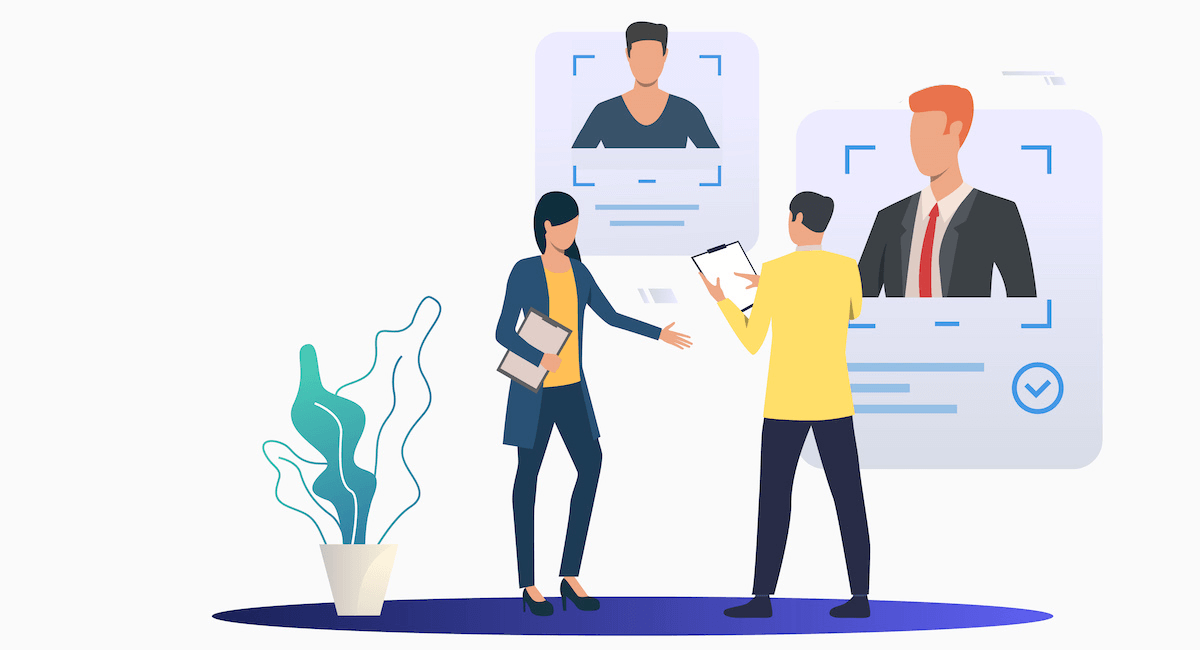
Types of Genomic Datasets Worked With
Look for candidates who have had exposure to various genomic datasets. Their ability to handle and understand different types of data can contribute significantly to their problem-solving and data analysis strategies.
Experience with Large-Scale Genomic Data Analysis
It's crucial to have someone with experience handling large volumes of genomic data, given the data-intensive nature of genomics. Insight into large-scale genomic data analysis will be a significant asset in any project.
Proficiency in Python and R Programming Languages
Python and R are two essential programming languages in genomics. These languages are commonly used in analyzing and interpreting genomic data, so having a firm grasp of these is paramount.
Familiarity with Bioinformatics Tools
Tools like Blast, Clustal Omega, or BioPython dramatically streamline the data analysis process in genomics. If your candidate is well versed in these tools, they can create accurate and efficient results.
Machine Learning Algorithms for Genomic Data Interpretation
Machine learning algorithms are becoming increasingly crucial in genomic data interpretation as they can find patterns and make predictions from incredibly complex data.
Approach Towards Cleaning and Preprocessing Genomic Data
To get valid and reliable results from any data analysis, one first needs to ensure that the data is clean and well-structured. This is just as true in genomics.
Challenges Associated With Storing and Managing Genomic Data
Genomic data are high-dimensional and large in volume. The way your candidate handles associated storage and management challenges speaks volumes about their problem-solving capabilities and organizational skills.
Integration and Analysis of Omics Data
Integrating and interpreting various omics data types—including genomics, proteomics, and transcriptomics—can lead to better understanding of biological mechanisms. Ask if the candidate has experience in this area.
Experience With Cloud Computing Platforms
Cloud computing platforms, like Amazon Web Services and Google Cloud, offer cost-effective and scalable solutions for analyzing and storing vast genomic data.
Data Security and Privacy Measures
Dealing with sensitive genomic data comes with the responsibility of ensuring its security and privacy. It's crucial to find an individual who understands this and takes it seriously.
Understanding of Population Genetics and Molecular Evolution
Leaders in the field of genomics have a comprehensive understanding of the field, including the principles of population genetics and molecular evolution.
Publications or Research in Genomic Data Science
A history of published research or continuing work in genomic data science demonstrates a candidate's dedication to their field and provides a concrete sample of their work.
Development of Novel Computational Approach in Genomics
Novel approaches to data analysis can lead to unexpected insights. A candidate who isn't afraid to think outside the box could bring unique perspectives to your team.
Experience in Statistical Genetic Analyses Execution
Statistical genetic analyses form the backbone of many genomic studies. Candidates should be familiar with designing and executing these types of analyses.
Handling of Missing or Inconsistent Data
Genetic data is often riddled with missing or inconsistent data points. Therefore, the way a candidate handles such issues could reflect their resourcefulness and critical thinking skills.
Tools Used for Genomic Data Visualization
Proper data visualization is key to communicating genomic data insights. It's an important and overlooked skill in a potential candidate.
Analysis of Data from Next-Gen Sequencing Technologies
Familiarity with RNA-seq, ChIP-seq, or exome sequencing data analysis can indicate a candidate's proficiency with advanced genomic technologies.
Projects Identifying Genetic Variants Associated with a Disease
Being able to use genomic data to identify disease-associated genetic variants signifies a deep understanding of genomics and its practical applications.
Experience With Genomic and Genetic Data Databases
Databases such as dbSNP and ClinVar are critical resources in genomics research. Candidates should be comfortable utilizing these databases.
Genetic Disease Modeling and Predictive Analytics
The ultimate goal of many genomics projects is to model genetic diseases and predict their occurrence. Experience in this area can be of immense value to your team.
Prescreening questions for Genomic Data Scientist
- Describe your experience with high-throughput sequencing data analysis.
- What bioinformatics tools and software are you most proficient in?
- Can you discuss your familiarity with genome assembly and annotation?
- What programming languages do you use for genomic data analysis?
- Describe your experience working with large genomic datasets.
- How do you handle missing or inconsistent data in genomic studies?
- Can you explain a project where you used machine learning techniques on genomic data?
- How do you stay current with the latest developments in genomics and bioinformatics?
- Describe your experience with cloud computing platforms for genomic data analysis.
- What types of genomic databases are you most familiar with?
- Can you discuss your experience with statistical analysis in genomics?
- Describe a challenging problem you encountered in a genomic project and how you solved it.
- What version control systems do you use for managing code in your projects?
- How do you ensure reproducibility in your genomic analyses?
- Can you explain the role of comparative genomics in your work?
- What experience do you have with gene expression analysis?
- How do you manage data privacy and security in genomic research?
- Describe your experience with collaborative projects in genomics.
- What methodologies do you use to integrate multi-omics data?
- Can you discuss your familiarity with population genetics analysis tools and techniques?
- What types of genomic datasets have you worked with in your previous roles?
- Can you describe your experience with large-scale genomic data analysis?
- What is your level of proficiency in Python and R programming languages?
- How familiar are you with bioinformatics tools such as Blast, Clustal Omega, or BioPython?
- Have you developed any machine learning algorithms for genomic data interpretation?
- What is your approach towards cleaning and preprocessing genomic data?
- In your past roles, how did you handle the challenges associated with storing and managing genomic data?
- Can you explain a project where you integrated and analysed different type of omics data (like proteomic, genomic, transcriptomic)?
- Do you have experience with cloud computing platforms, such as Amazon Web Services or Google Cloud, for genomic data analysis?
- How would you ensure data security and privacy while dealing with sensitive genomic information?
- Can you describe your understanding of population genetics and molecular evolution?
- Do you have any publications or research in genomic data science?
- Describe one instance where you developed or applied a novel computational approach to solve a challenging problem in genomics.
- Do you have any experience in the development and execution of statistical genetic analyses?
- How do you handle missing or inconsistent data in a genome sequence analysis?
- What tools do you typically use for the visualization of genomic data?
- Can you explain how you would analyze data from next-gen sequencing technologies: RNA-seq, ChIP-seq, or exome sequencing?
- Can you describe a project where you identified genetic variants associated with a disease using genomic data?
- Have you worked with any databases for genomic and genetic data, such as dbSNP and ClinVar?
- Do you have any experience working with genetic disease modeling and predictive analytics?
Interview Genomic Data Scientist on Hirevire
Have a list of Genomic Data Scientist candidates? Hirevire has got you covered! Schedule interviews with qualified candidates right away.