Prescreening Questions: Essential Strategy for Successful Recruitment Machine Learning Governance Analyst
Machine Learning (ML) Governance is an increasingly pivotal concept in today's data-driven world, especially with ever-growing compliance requirements. This article delves into 20 essential prescreening questions that should be asked while hiring a Machine Learning specialist or executive. We will dig deeper into each question, outlining the relevance and potential answers that can help assess a candidate's competency adequately.
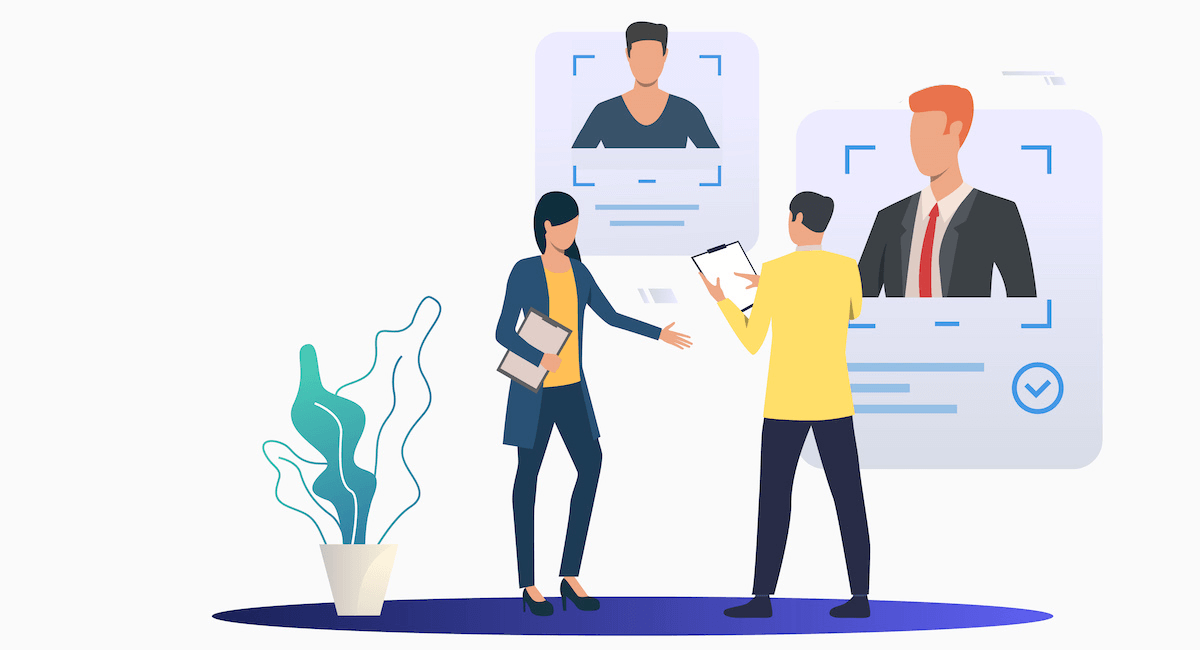
What is your understanding of Machine Learning Governance?
Machine Learning governance implies the robust management, monitoring, and control over ML models within a company. An ideal candidate will understand it as a necessarily proactive process that ensures data quality, data privacy, model accuracy and performance, and mitigates the potential risk associated with the deployment of ML models.
How would you handle a situation where a machine learning model is not providing the desired results?
This question assesses a candidate's problem-solving strategy. The notion of retraining the model, verifying data quality, or running diagnostics to identify issues with the model are possible answers that may demonstrate their pragmatic approach.
Can you explain your experience with data privacy and compliance regulations such as GDPR and CCPA?
In today's era of data sensitivity, understanding data privacy laws like GDPR and CCPA is paramount for anyone dealing with data. Candidates should demonstrate knowledge of these regulations and narrate how they adhered to them in previous roles.
How have you ensured that machine learning models adhere to data ethics principles in your past roles?
This question checks the candidate's ethical knowledge, and they should indicate the process of maintaining transparency, privacy, security, and fairness of data in their past projects.
Can you describe your familiarity with data preparation and analysis?
Proficiency in data preparation and analysis form the backbone of machine learning. Candidates should talk about their experience with handling missing data, data cleaning, and other facets of data preprocessing that ensure efficient model training.
Do you have experience implementing auditing and monitoring frameworks in machine learning?
Candidates must detail their expertise in implementing auditing and monitoring frameworks to check the performance, validity, and fairness of ML models.
Can you talk about any machine learning project where you were involved in making key decisions?
This question provides an opportunity for the candidate to showcase their decision-making skills in a real-life scenario. It is essential to listen to how they evaluated alternatives and calculated risks before making the critical decisions.
What kind of machine learning platforms do you have experience working with?
An experienced candidate should possess familiarity with multiple ML platforms (like TensorFlow, Keras, PyTorch) and should articulate the pros and cons of each.
How can you ensure the validity of data used for training machine learning models?
Here, candidates should discuss strategies for data preprocessing, data auditing, and data cleaning to ensure data validity.
What is your experience with risk assessment in machine learning?
The candidate should showcase prior experience in assessing risks associated with bias, data leakage, and model stability in machine learning.
Have you ever faced any ethical dilemmas during your projects and how did you handle it?
This question not only tests the candidate's ethical stand but also their quick-thinking skills when they are up against an ethical quandary.
Can you describe your familiarity with various machine learning algorithms?
From linear regression to neural networks, the candidate should demonstrate proficiency with several machine learning algorithms and their practical implications.
Do you have experience with machine learning model lifecycle management?
Candidates should elaborate on their experiences managing different phases of an ML model's lifecycle, including data collection, preprocessing, modeling, validation, deployment, monitoring, and updating.
How would ensure transparency when a machine learning model is utilized?
The answer could involve strategies for documenting assumptions, inputs, feature importance, and model performances or deploying interpretable machine learning methods.
Do you have any experience with cross-functional team collaboration in an ML project?
Machine learning projects often involve experts from different domains for successful execution. Candidates should detail their experiences in such cross-functional collaborations.
How familiar are you with the concept of Continuous Improvement in machine learning models?
This tests whether the candidate is aware of the cyclic and evolving nature of machine learning models and whether they can iteratively improve models based on new data or feedback.
What is your understanding of machine learning model bias and fairness?
An effective candidate should be knowledgeable about different types of bias and methods to ensure fairness during model training and prediction.
Can you explain your experience in troubleshooting and fine-tuning machine learning models?
Candidates should discuss their strategies for improving model performance through hyperparameter tuning, feature selection, or feature engineering.
Can you describe a scenario where you had to present the results of a complex machine learning model to a non-technical audience?
Every ML practitioner needs to explain complex models to stakeholders who may not be technically savvy. Candidates should showcase their communication skills in making complicated concepts easily graspable.
How would you keep abreast with the latest developments and trends in machine learning?
This is to ensure if a candidate is inclined towards continued learning. They should talk about their sources for news, updates and how they incorporate this knowledge into their ongoing work.
Prescreening questions for Machine Learning Governance Analyst
- What is your understanding of Machine Learning Governance?
- How would you handle a situation where a machine learning model is not providing the desired results?
- Can you explain your experience with data privacy and compliance regulations such as GDPR and CCPA?
- How have you ensured that machine learning models adhere to data ethics principles in your past roles?
- Can you describe your familiarity with data preparation and analysis?
- Do you have experience implementing auditing and monitoring frameworks in machine learning?
- Can you talk about any machine learning project where you were involved in making key decisions?
- What kind of machine learning platforms do you have experience working with?
- How can you ensure the validity of data used for training machine learning models?
- What is your experience with risk assessment in machine learning?
- Have you ever faced any ethical dilemmas during your projects and how did you handle it?
- Can you describe your familiarity with various machine learning algorithms?
- Do you have experience with machine learning model life cycle management?
- How would ensure transparency when a machine learning model is utilized?
- Do you have any experience with cross-functional team collaboration in an ML project?
- How familiar are you with the concept of Continuous Improvement in machine learning models?
- What is your understanding of machine learning model bias and fairness?
- Can you explain your experience in troubleshooting and fine-tuning machine learning models?
- Can you describe a scenario where you had to present the results of a complex machine learning model to a non-technical audience?
- How would you keep abreast with the latest developments and trends in machine learning?
Interview Machine Learning Governance Analyst on Hirevire
Have a list of Machine Learning Governance Analyst candidates? Hirevire has got you covered! Schedule interviews with qualified candidates right away.