Unlocking the Power of Prescreening: Crucial Questions to Ask for Successful Nano Data Scientist Role
In the rapidly evolving realm of technology, Nano Data Science is a burgeoning field, intersecting two fascinating areas - nanotechnology and data science. With a myriad of practical applications and promising potential, Nano Data Scientists are the pioneers tasked with leveraging tiny tech to unravel large data mysteries. This article will explore some prescreening questions to gauge the understanding and qualifications of aspiry Nanodata Scientists.
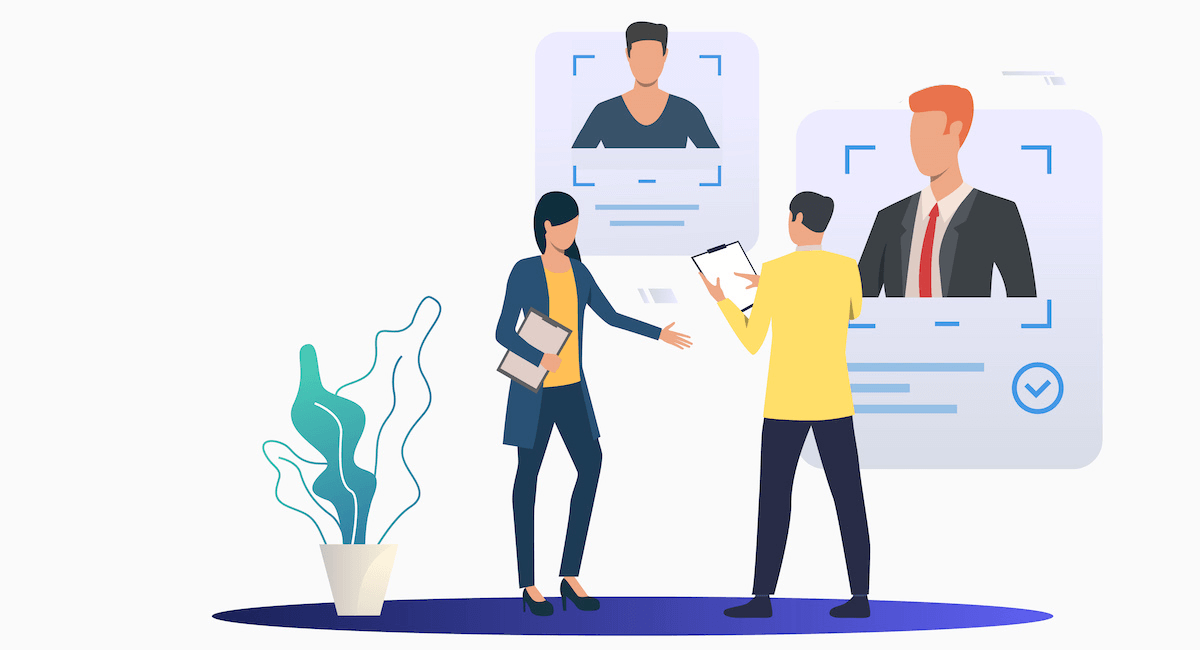
Understanding of Nanotechnology in Data Science
Nanotechnology's integration into data science represents a leap forward for the technical world. Nano Data Science doesn't simply signify working with micro-sized tech. Instead, it encompasses operating on nanoscales to enhance data storage, processing, and security among other pursuits.
Qualifications for a Nano Data Scientist Role
As the frontier of a relatively new field, a Nano Data Scientist needs a robust foundation in data science and nanotechnology, along with a natural curiosity and an innovative spirit. Strong programming skills, statistical knowledge, and a history of relevant research are crucial.
Explaining Nano Data Science to a Layperson
Nano Data Science could be narratively related to playing a complex jigsaw puzzle, where every piece represents a data point. The nanotechnology here acts as a magnifying glass to see and organize these pieces more accurately and effectively.
Practical Applications of Nanotechnology in Data Science
When nanotechnology meets data science, we witness advancements in areas like healthcare, where nanobots parse through big data to provide personalised medications. It also influences cybersecurity, data storage, and processing speeds, pushing the technological boundaries.
Proficient Programming Languages
Proficient programming languages for Nano Data Scientists primarily include Python and R. Mastery over these languages can significantly impact their capability to analyze complex datasets and build effective models.
Problem Solving Approach
Problem solving in this field requires a systematic and analytical approach. Ideally, issues are broken down into smaller parts, assumptions are questioned, and data is scrutinised. Often, creativity in combining existing solutions can yield the best results.
Examples of Nano Data Science Projects
Real world usage of Nano Data Science could be seen in projects focusing on improving patient care in healthcare, enhancing data encryption in cybersecurity, and developing high-capacity, energy-efficient data storages.
Recent Advancements in Nanotechnology for Data Science
Recent advancements like carbon nanotube storage devices promise to revolutionize data storage, enhancing capacity and reducing energy consumption. Such innovations have significant implications for data science, particularly in handling big data.
Ensuring Data Accuracy and Quality
Data quality management is ensured through rigorous data cleaning and preprocessing methods. In addition, managing potential bias and carefully choosing data sources also play a crucial role.
Experience with Machine Learning
Machine Learning plays an integral role in Nano Data Science, used for building predictive models that can analyze datasets and predict outcomes with high accuracy.
Familiarity with Statistical Analysis
An intrinsic understanding of statistical analysis is crucial as it forms the backbone of data interpretation, allowing for informed insights and decision making.
Handling Large Datasets
Handling large datasets comes with challenges such as efficient storage, meticulous cleaning, and accurate analysis. It requires strategic planning, robust technology, and patience.
Data Visualization and Reporting
Effective data visualization allows complex data to be understood easily, thereby enabling well-informed decisions. Reporting in a clear and concise manner is also significant for conveying crucial insights.
Staying Updated with Nano Data Science Trends
Continuous learning and curiosity are paramount for staying updated in this rapidly evolving field. This could involve attending conferences, reading research papers, and participating in relevant communities and forums.
Data Influencing Decisions and Strategies
Every dataset harbors a galaxy of insights waiting to be uncovered. Analyzing these data trails critically can influence strategic decision-making and shape the direction of various projects.
Writing Algorithms for Data Analysis
Writing algorithms is central to data processing and analysis. It involves understanding the problem, planning the approach, and creatively crafting an efficient solution that meets the requirement.
Preferred Tools or Software
Preferred tools might include Python, R, Jupyter notebooks, Tableau for visualization, and Git for version control. The selection usually depends on the problem at hand and personal preference.
Publications and Research Experience
Having researched or published in the field showcases one's depth of understanding and familiarity with cutting-edge trends.
Explaining Technical Findings to a Non-Technical Stakeholder
Explaining technical findings to non-technical stakeholders is an art, reducing complexity and translating it into understandable terms using metaphors and storytelling.
Challenges in Nano Data Science and Overcoming Them
The dynamic nature of this field presents several challenges including proper data handling, maintaining security, and managing energy consumption. These can be overcome through continuous learning, innovation, and collaboration.
Prescreening questions for Nano Data Scientist
- What is your understanding of nanotechnology in data science?
- What qualifications do you have that make you fit for the role of a Nano Data Scientist?
- How would you explain Nano Data Science to someone with no technical background?
- Can you describe some practical applications of nanotechnology in the field of data science?
- What programming languages are you most proficient in, and how does this impact your work as a Nano Data Scientist?
- What is your approach to problem-solving when you encounter an issue in your data analysis or modeling?
- Can you provide examples of projects in which you used Nano Data Science?
- Can you discuss one of the recent advancements in nanotechnology and its implications for data science?
- How do you ensure data accuracy and quality in your work?
- Describe your experience with machine learning and its applications in Nano Data Science.
- How familiar are you with statistical analysis and data-driven problem solving?
- Explain how you handle large datasets and the challenges associated with them.
- What experience do you have with data visualization and creating comprehensive reports?
- How do you stay updated with the current trends and advancements in Nano Data Science?
- Can you describe a time when you used data to develop a strategy or influence a decision?
- How comfortable are you with writing algorithms and implementing them into your data analysis?
- Are there any specific tools or software that you prefer using in your work as a Nano Data Scientist?
- Do you have any publications or research experience in the field of Nano Data Science?
- How would you approach a situation where you need to explain your technical findings to a non-technical stakeholder?
- What do you think are the biggest challenges in Nano Data Science and how do you propose to overcome them?
Interview Nano Data Scientist on Hirevire
Have a list of Nano Data Scientist candidates? Hirevire has got you covered! Schedule interviews with qualified candidates right away.