Prescreening Questions to Ask Neuro-Symbolic AI Training Data Curator
Are you about to dive into the world of neuro-symbolic AI? Whether you're a hiring manager seeking the right candidate or an enthusiast looking to gauge your know-how, understanding the right questions to ask is critical. Neuro-symbolic AI is a fascinating blend of symbolic reasoning and neural networks, and its success relies heavily on quality data, effective methodologies, and up-to-date practices. Ready to explore? Let's get started!
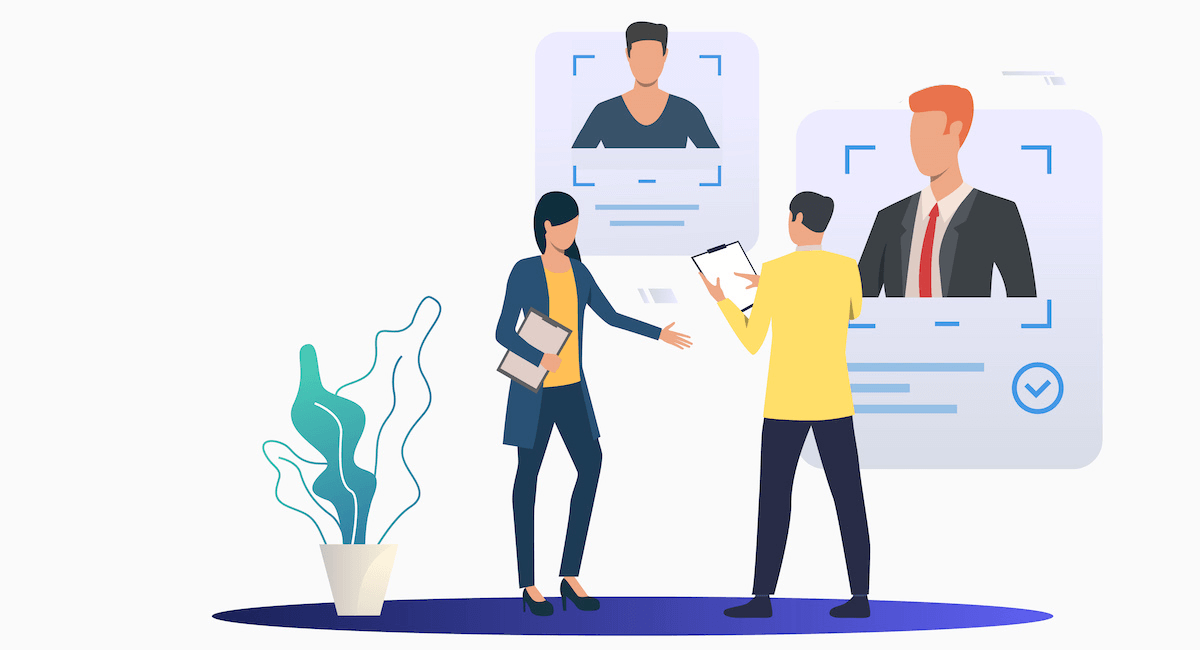
Can you describe your experience with neuro-symbolic AI?
Imagine you're sitting across from a candidate, brimming with curiosity about their journey in neuro-symbolic AI. This question sets the stage. Look for detailed and varied experiences, as this showcases their depth of knowledge. Have they tackled real-world problems? Or perhaps developed innovative solutions using this hybrid approach?
What methodologies have you used for annotating AI training datasets?
Annotation might sound mundane, but it's the lifeblood of effective AI. How someone approaches this task can reveal a lot about their rigour and innovative thinking. Are they traditionalists, sticking to tried-and-true methods? Or do they prefer cutting-edge techniques?
How do you ensure data quality and consistency in large datasets?
Data consistency is like the harmony in a symphony. Without it, the result is chaos. Ask them to describe the tools and checks they use to maintain pristine data quality. Are they proactive or reactive in detecting and fixing discrepancies?
What techniques do you use to handle noisy or unstructured data?
Noisy data is the static in a podcast you're trying to enjoy. It demands clever tricks and techniques to filter out the noise and refine the content. Do they have a toolbox full of filtering algorithms or machine learning techniques to tackle this?
Have you worked with both symbolic reasoning and neural networks?
Neuro-symbolic AI lives at the intersection of symbolic reasoning and neural networks. Someone experienced in both areas can fluently switch between analyzing high-level rules and crunching numbers. This versatility can be the difference between an okay model and a stellar one.
How do you integrate symbolic and neural components in AI models?
Integration isn’t just a buzzword. It’s an art form. Listen for insights into their creative process and the architecture they use. How do they seamlessly blend the rule-based reasoning of symbolic AI with the pattern recognition prowess of neural networks?
Can you give examples of projects where you've curated training data for AI models?
Real-world examples? Yes, please! Let’s hear about the nitty-gritty. Which projects have they found challenging yet rewarding? These stories can illuminate not just their technical prowess but also their problem-solving skills and tenacity.
How do you approach the challenge of bias in training data?
Bias is like a hidden trap in a maze. It’s sneaky and can trip up even seasoned experts. Do they have strategies for identifying and mitigating bias? Ethical AI isn’t just desirable; it’s crucial.
What tools and technologies are you proficient with for data curation?
Tool proficiency can streamline workflows and boost productivity. Are they well-versed in platforms like TensorFlow or PyTorch? Or maybe they’ve got a knack with data annotation tools like Labelbox?
How do you validate the effectiveness of the training data you've curated?
Validation techniques are akin to quality control in manufacturing. Without them, you're flying blind. Do they use cross-validation, bootstrapping, or maybe newer methods? This reveals their commitment to robust, reliable models.
What are the common pitfalls in creating training datasets for neuro-symbolic AI?
It's always good to learn from others' mistakes, isn't it? Discussing common pitfalls can shed light on their experience and foresight. Can they foresee data bottlenecks or annotation inconsistencies?
How do you stay updated with the latest trends in neuro-symbolic AI?
You wouldn’t trust a doctor who doesn’t keep up with medical advancements, right? The same applies to AI experts. Are they avid readers of journals, or do they attend conferences and webinars to stay on the cutting edge?
Can you describe a time when you had to solve a complex data-related problem?
This is their chance to be the hero of their own story. Complex problems are inevitable, but how they tackle them is what sets them apart. Listen for creativity, persistence, and, of course, a successful outcome.
How do you manage and document metadata for training datasets?
Metadata management might not sound glamorous, but it’s the backbone of organized data. Proper documentation ensures everyone’s on the same page and can save hours down the line. How meticulous are they with metadata?
What is your approach to collaborating with data scientists and engineers?
AI work is rarely solo. It’s more like a team sport where collaboration is key. Are they good communicators? Flexible and open to feedback? Team dynamics can make or break a project.
How do you prioritize tasks when curating datasets with tight deadlines?
Deadlines can be pressure cookers. The real test is how they handle the heat. Do they have a battle-tested prioritization method to ensure quality doesn't slip even when time is tight?
What strategies do you employ to scale data curation processes?
Scale can make or break an AI project. Can they think big and adapt processes to handle larger data volumes without compromising quality? Efficiency and scalability are the gold standards.
Can you explain the role of ontologies in neuro-symbolic AI?
Ontologies help AI models understand the world in a structured way, like a librarian organizing books by genre. How adept are they at crafting and integrating ontologies to enrich AI reasoning?
How do you handle version control for training datasets?
Ever tried working on an outdated file? It’s a nightmare! Version control ensures everyone’s in sync. Their method tells you if they’re organized and savvy with tools like Git or other version control systems.
What are your thoughts on the future of neuro-symbolic AI?
Let them speculate a bit! Their vision for the future can reveal their passion and insight into the evolving landscape of neuro-symbolic AI. Are they optimistic, cautious, or perhaps somewhere in between?
Prescreening questions for Neuro-Symbolic AI Training Data Curator
- Can you describe your experience with neuro-symbolic AI?
- What methodologies have you used for annotating AI training datasets?
- How do you ensure data quality and consistency in large datasets?
- What techniques do you use to handle noisy or unstructured data?
- Have you worked with both symbolic reasoning and neural networks?
- How do you integrate symbolic and neural components in AI models?
- Can you give examples of projects where you've curated training data for AI models?
- How do you approach the challenge of bias in training data?
- What tools and technologies are you proficient with for data curation?
- How do you validate the effectiveness of the training data you've curated?
- What are the common pitfalls in creating training datasets for neuro-symbolic AI?
- How do you stay updated with the latest trends in neuro-symbolic AI?
- Can you describe a time when you had to solve a complex data-related problem?
- How do you manage and document metadata for training datasets?
- What is your approach to collaborating with data scientists and engineers?
- How do you prioritize tasks when curating datasets with tight deadlines?
- What strategies do you employ to scale data curation processes?
- Can you explain the role of ontologies in neuro-symbolic AI?
- How do you handle version control for training datasets?
- What are your thoughts on the future of neuro-symbolic AI?
Interview Neuro-Symbolic AI Training Data Curator on Hirevire
Have a list of Neuro-Symbolic AI Training Data Curator candidates? Hirevire has got you covered! Schedule interviews with qualified candidates right away.