Prescreening Questions to Ask Quantum-Enhanced Drug Interaction Predictor
Are you diving into the captivating world of quantum computing to revolutionize drug interaction predictions? Before you take the plunge, it's essential to ask the right questions. This guide walks you through essential queries that will help you gauge the robustness, reliability, and overall effectiveness of quantum solutions out there. Ready to unlock the mysteries? Let's get started!
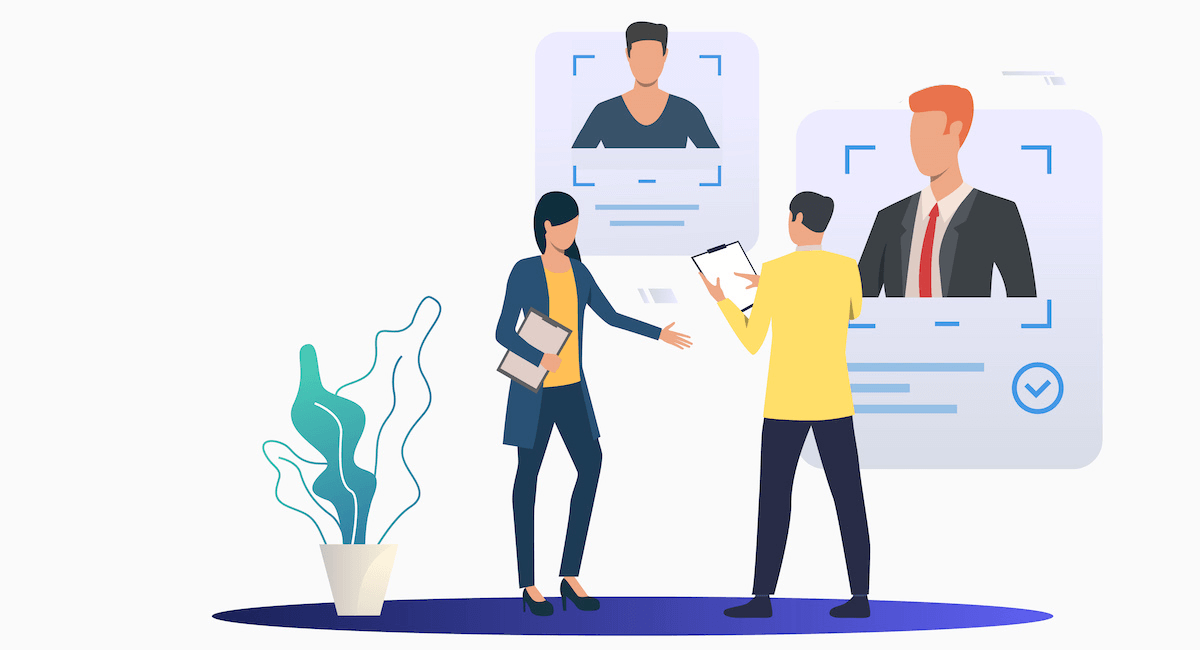
What specific quantum algorithms do you implement for drug interaction prediction?
At the heart of any quantum computing solution lies its algorithms. Different algorithms offer varying capabilities, and it’s important to know which ones are used for drug interaction predictions. Do they leverage variational quantum eigensolvers (VQE) or quantum approximate optimization algorithms (QAOA)? This choice can affect the accuracy and speed of the predictions.
How does your system handle large datasets and ensure scalability?
Handling large datasets is crucial for any predictive model. The system should be designed to not just manage but also scale efficiently. How does it ensure that as the dataset grows, the speed and performance don’t degrade? Does the solution incorporate advanced data compression techniques or parallel processing capabilities?
Can you describe the types of quantum hardware you support?
Quantum computing is still evolving, and various types of hardware exist. Understanding the hardware types—whether it's superconducting qubits, trapped ions, or topological qubits—can give insights into the system's capabilities. Each type has its own advantages and challenges, and knowing this can help you align your needs better.
What is the accuracy rate of your drug interaction predictions?
Accuracy is a deal-breaker when it comes to predictive models. It’s essential to know the accuracy rate and how it compares to existing methods. Are the predictions consistently reliable? The last thing you want is to base critical decisions on unreliable data.
How do you ensure the reliability and robustness of your model outputs?
Reliability and robustness are non-negotiable. How does the system ensure these aspects? Does it undergo routine stress testing and validation? Understanding the measures in place gives you confidence that the predictions can be trusted.
What kind of validation process do you use for your predictions?
Validation processes are key to maintaining accuracy and reliability. Does the system use cross-validation or holdout methods? What benchmarks or datasets are utilized to validate the predictions? This ensures that the predictions are not just theoretically sound but also practically reliable.
How do you integrate classical computing with quantum computing in your system?
Quantum computing is powerful but still needs classical computing to handle some tasks. How does the system integrate these two realms? Is there a seamless flow of data between quantum and classical nodes? This hybrid approach can enhance the capabilities and efficiency of the solution.
What potential drug interactions have you successfully predicted so far?
Evidence speaks louder than words. Knowing the drug interactions already predicted by the system adds credibility. Past successful predictions can serve as testimonials to the system’s effectiveness and reliability.
How does your solution compare to traditional drug interaction prediction methods?
The whole idea of embracing new technology is to surpass existing limitations. How does this quantum computing solution fare against traditional methods? Are the predictions faster, more accurate, or cost-effective? This comparison can help justify the shift to quantum solutions.
What is the typical turnaround time for running a prediction?
Time is of the essence in drug discovery and healthcare. Knowing the typical turnaround time for predictions can help manage expectations and plan better. Is it a matter of seconds, minutes, or hours? Faster predictions can drastically cut down development times.
How do you handle noise and error correction in your quantum computations?
Quantum systems are prone to noise and errors, which can affect the quality of computations. How does the system tackle these challenges? Are there any specific error correction codes or redundancy measures in place? Effective noise management is essential for robust predictions.
Can your system identify previously unknown interactions between drugs?
One of the exciting potentials of quantum computing is uncovering previously unknown interactions. Can the system identify these novel interactions? This capability can lead to groundbreaking discoveries and significantly impact drug development.
What security measures are in place to protect sensitive data?
Data security is paramount, especially when dealing with sensitive health information. What security protocols are in place to protect this data? Is the data encrypted, and are there access controls? Ensuring robust security measures can prevent unauthorized access and data breaches.
Do you offer customization options for specific user requirements?
Every research project or organization may have unique needs. Does the system offer customization options to cater to these specific requirements? Customizable solutions can provide more relevant predictions and better user satisfaction.
How often do you update your models and algorithms to incorporate new scientific knowledge?
The field of drug interactions is ever-evolving. Frequent updates to models and algorithms are important to incorporate the latest scientific knowledge. How often does the system get updated? Regular updates ensure that the predictions are as accurate and relevant as possible.
What kind of expertise or training is required to use your platform effectively?
The usability of the platform is crucial. Does it require specialized quantum computing knowledge, or is it user-friendly enough for people with basic computational biology backgrounds? Knowing this can help assess the resource and training needs for your team.
How do you address the computational complexities inherent in quantum mechanics?
Quantum mechanics is inherently complex. How does the system manage these complexities? Are there simplification techniques or abstractions in place to make the computations more manageable? Tackling these complexities can lead to more efficient and reliable predictions.
Can you integrate your solution with existing pharmaceutical research workflows?
Integration with existing workflows can make or break the usability of a new solution. Can the quantum computing solution be seamlessly integrated into current pharmaceutical research workflows? This can ensure smoother transitions and better adoption rates.
What are the primary benefits your solution offers over current market alternatives?
Why choose this solution over others? Understanding the unique benefits, whether it's faster predictions, higher accuracy, or cost-effectiveness, can help make a more informed decision. The primary benefits should align with your organization's goals and challenges.
What partnerships or collaborations are you engaged in to advance this technology?
Collaborations can accelerate advancements and provide additional credibility. Is the solution part of any significant partnerships or collaborations? These can provide access to cutting-edge research, resources, and expertise, enhancing the overall capabilities of the system.
Prescreening questions for Quantum-Enhanced Drug Interaction Predictor
- What specific quantum algorithms do you implement for drug interaction prediction?
- How does your system handle large datasets and ensure scalability?
- Can you describe the types of quantum hardware you support?
- What is the accuracy rate of your drug interaction predictions?
- How do you ensure the reliability and robustness of your model outputs?
- What kind of validation process do you use for your predictions?
- How do you integrate classical computing with quantum computing in your system?
- What potential drug interactions have you successfully predicted so far?
- How does your solution compare to traditional drug interaction prediction methods?
- What is the typical turnaround time for running a prediction?
- How do you handle noise and error correction in your quantum computations?
- Can your system identify previously unknown interactions between drugs?
- What security measures are in place to protect sensitive data?
- Do you offer customization options for specific user requirements?
- How often do you update your models and algorithms to incorporate new scientific knowledge?
- What kind of expertise or training is required to use your platform effectively?
- How do you address the computational complexities inherent in quantum mechanics?
- Can you integrate your solution with existing pharmaceutical research workflows?
- What are the primary benefits your solution offers over current market alternatives?
- What partnerships or collaborations are you engaged in to advance this technology?
Interview Quantum-Enhanced Drug Interaction Predictor on Hirevire
Have a list of Quantum-Enhanced Drug Interaction Predictor candidates? Hirevire has got you covered! Schedule interviews with qualified candidates right away.