Prescreening Questions to Ask Quantum Machine Learning Fairness and Bias Auditor
Are you navigating the exciting world of quantum machine learning and wondering about the right prescreening questions to ask potential candidates? These questions can not only unravel their expertise but also provide insights into their problem-solving abilities, ethical considerations, and staying current with technological advancements. By the end of this article, you'll have a comprehensive list of questions to help you assess the right talent effectively.
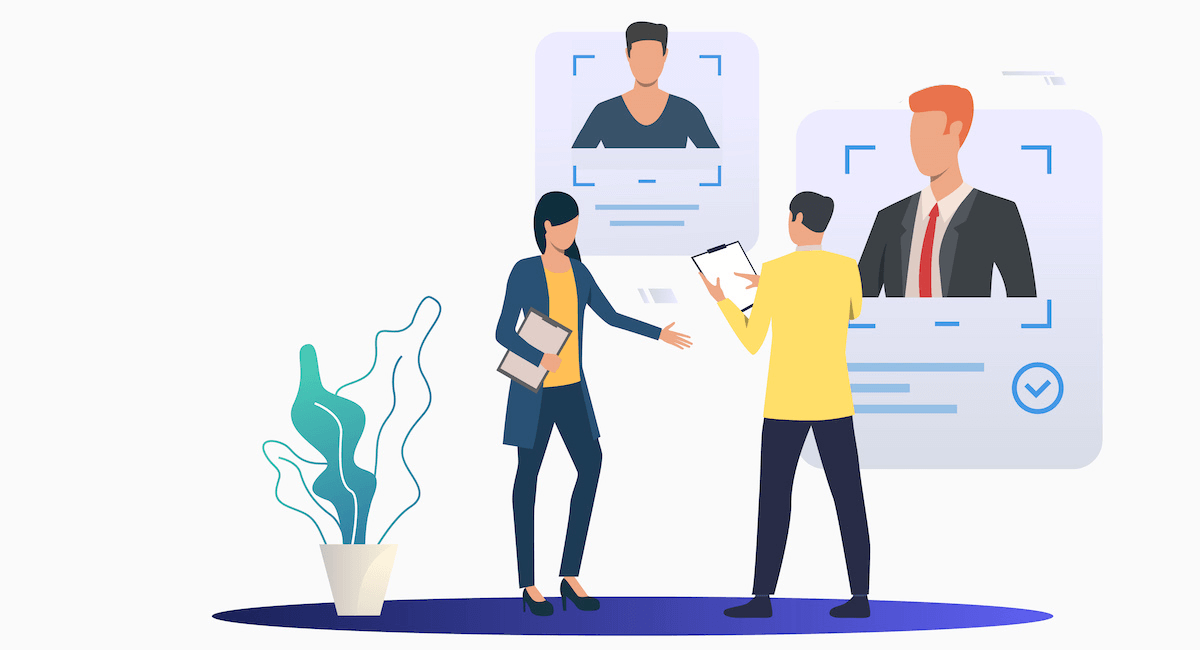
What experience do you have in working with quantum algorithms and their application to machine learning?
Diving deep into this question can give you a clear picture of the candidate's hands-on experience. Have they worked on actual quantum algorithms, or is their knowledge primarily theoretical? Look for specific projects or papers they've worked on, which can reflect their practical understanding and proficiency.
How do you approach identifying and mitigating bias in quantum machine learning models?
Bias in machine learning is like a sneaky shadow - it’s there, even if you can't see it. A solid approach to identifying and mitigating bias involves various techniques. Do they rely on statistical methods, or do they use advanced frameworks? Understanding their methodology can tell you a lot about their problem-solving skills.
Can you provide examples of projects where you've audited machine learning models for fairness?
Real-world examples speak volumes. Hearing about specific projects where they’ve audited models helps gauge their experience and effectiveness. Were they successful in identifying biases? What steps did they take to rectify them? These details will shed light on their practical know-how.
What tools and frameworks do you prefer for auditing quantum machine learning models?
The choice of tools and frameworks can significantly impact the efficiency and thoroughness of an audit. Do they prefer open-source tools, or do they rely on proprietary software? Asking this question helps understand their practical skill set and the technologies they are comfortable with.
How do you stay current with advancements in quantum computing and machine learning?
The tech world is ever-evolving, and staying updated is crucial. Do they partake in online courses, attend conferences, or follow specific journals? Their approach to continuous learning can indicate their commitment to staying at the cutting edge of technology.
What is your understanding of the ethical implications of bias in machine learning systems?
Ethics in machine learning is like the North Star; it guides the integrity of the work. Understanding the ethical implications of bias reflects a candidate's depth of knowledge and conscientiousness. Are they familiar with how biases can affect different populations?
Describe your experience with statistical analysis in the context of detecting bias in data.
Statistical analysis is a fundamental tool in the data scientist’s toolkit. The candidate's skill in leveraging statistical techniques to detect bias can be a game-changer. Are they proficient in methods like hypothesis testing or regression analysis?
How do you handle situations where biases are detected in historical data used for training models?
Historical data often carries historical biases. How do they rectify this situation? Do they employ techniques like data balancing or synthetic generation? Their approach can reveal their problem-solving skills and ethical considerations.
What steps would you take to ensure the fairness of a quantum machine learning model from the outset?
Starting on the right foot ensures fewer headaches down the road. What methodologies do they employ to incorporate fairness from the beginning? Their strategy could include diverse data collection, regular audits, or specific fairness metrics.
How do you validate the results of a quantum machine learning audit for accuracy and reliability?
Validation is like the quality assurance of machine learning. Ensuring the accuracy and reliability of an audit involves cross-validation, robustness checks, and possibly third-party reviews. Their method of validation can highlight their attention to detail and thoroughness.
What strategies do you use to explain complex quantum algorithms to stakeholders without a technical background?
Explaining quantum algorithms to non-technical stakeholders is like translating a foreign language. Do they use analogies, visual aids, or simplified explanations? Their ability to communicate complex ideas in a simple manner is crucial for collaborations and decision-making.
Describe a challenging scenario you faced when auditing a machine learning model for bias and how you resolved it.
Challenges often bring out the best in professionals. Hearing about a specific challenging scenario and their resolution approach provides insight into their problem-solving skills, perseverance, and resourcefulness.
How familiar are you with regulatory standards and guidelines regarding AI bias and fairness?
Compliance with regulatory standards ensures the ethical deployment of AI models. Are they up to date with the latest guidelines from organizations like IEEE or industry-specific standards? Their familiarity can indicate their commitment to ethical practices.
What methods do you use to benchmark the fairness of quantum machine learning models?
Benchmarking is essential for assessing relative fairness. Do they compare models against industry standards or use statistical measures? Their choice of benchmarking methods can reveal their analytical rigor and comparative skills.
How would you integrate fairness checks into the lifecycle of a quantum machine learning project?
Continuous fairness checks ensure that biases are addressed throughout the project lifecycle. Their approach to integrating these checks can provide insights into their project management skills and commitment to fairness.
What role do you believe transparency plays in ensuring the fairness of machine learning models?
Transparency is like lifting the curtain off machine learning models. Do they believe in open-source models, transparent algorithms, or clear communication about model behavior? Their perspective on transparency can highlight their ethical commitment.
Can you discuss your experience with quantum data encoding techniques and their impact on model fairness?
Quantum data encoding is a unique challenge with significant implications on model fairness. Do they have experience with techniques like amplitude encoding or basis encoding? Understanding their expertise can reflect their depth in quantum-specific tasks.
How do you approach cross-validation and testing to identify hidden biases in quantum machine learning models?
Cross-validation is like the detective work of model testing. Their approach to cross-validation and testing can reveal hidden biases that might not be apparent initially. Are they proficient with k-fold cross-validation or other sophisticated techniques?
What kind of partnerships or collaborations have you been involved in to enhance your auditing capabilities?
Collaborations often bring diverse perspectives and expertise. Have they worked with academic institutions, industry experts, or non-profits? Their collaborative experiences can enhance their auditing capabilities significantly.
How do you assess the impact of model retraining on fairness and bias mitigation in quantum machine learning systems?
Retraining models is essential for continuous improvement, but it’s not without its challenges. Their approach to assessing the impact of retraining on fairness can provide insights into their long-term strategy for bias mitigation.
Prescreening questions for Quantum Machine Learning Fairness and Bias Auditor
- What experience do you have in working with quantum algorithms and their application to machine learning?
- How do you approach identifying and mitigating bias in quantum machine learning models?
- Can you provide examples of projects where you've audited machine learning models for fairness?
- What tools and frameworks do you prefer for auditing quantum machine learning models?
- How do you stay current with advancements in quantum computing and machine learning?
- What is your understanding of the ethical implications of bias in machine learning systems?
- Describe your experience with statistical analysis in the context of detecting bias in data.
- How do you handle situations where biases are detected in historical data used for training models?
- What steps would you take to ensure the fairness of a quantum machine learning model from the outset?
- How do you validate the results of a quantum machine learning audit for accuracy and reliability?
- What strategies do you use to explain complex quantum algorithms to stakeholders without a technical background?
- Describe a challenging scenario you faced when auditing a machine learning model for bias and how you resolved it.
- How familiar are you with regulatory standards and guidelines regarding AI bias and fairness?
- What methods do you use to benchmark the fairness of quantum machine learning models?
- How would you integrate fairness checks into the lifecycle of a quantum machine learning project?
- What role do you believe transparency plays in ensuring the fairness of machine learning models?
- Can you discuss your experience with quantum data encoding techniques and their impact on model fairness?
- How do you approach cross-validation and testing to identify hidden biases in quantum machine learning models?
- What kind of partnerships or collaborations have you been involved in to enhance your auditing capabilities?
- How do you assess the impact of model retraining on fairness and bias mitigation in quantum machine learning systems?
Interview Quantum Machine Learning Fairness and Bias Auditor on Hirevire
Have a list of Quantum Machine Learning Fairness and Bias Auditor candidates? Hirevire has got you covered! Schedule interviews with qualified candidates right away.