Unlocking Success: Essential Prescreening Questions to Ask Trustworthy AI Engineer for Effective Hiring
During the prescreening phase for a role that requires proficiency in artificial intelligence, it is paramount to ask pointed questions that will help you assess the candidate's abilities, ethics, innovations and comprehension of the pertinent legal requirements. These questions play a crucial role in determining the suitability of a potential hire for the AI-related tasks ahead.
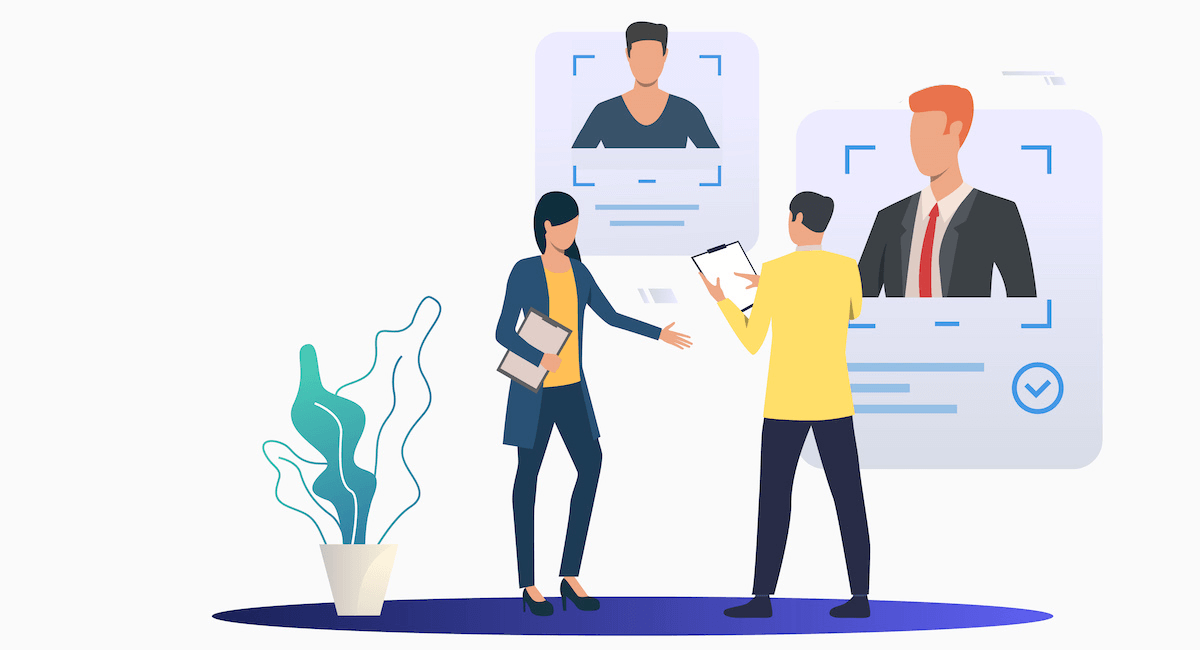
Experience with programming languages commonly used in AI
Having knowledge of AI-related programming languages is a requisite for anyone wishing to delve into AI. Python, Java, and R are top on the list. Asking a prospective hire about their experience with these languages gives you an insight into their knack for programming as it relates to AI.
Handling bias in machine learning models
Artificial Intelligence is only as good as the fairness of the data sets fed into it. Inasmuch as machine learning technologies are great at predicting patterns, their ability to do so in an unbiased way is paramount. Evaluating how a candidate handles bias in Machine Learning models is thus crucial.
Implementation of AI ethics and fairness
Fairness and ethics in AI is a rapidly growing concern. Applicants should be able to detail instances where they suggested or implemented fairness in their AI projects. This gives you a picture of how they approach this critical issue.
Familiarity with AI data usage regulations
AI ethics is not only about fairness; it also entails adherence to the legal provisions governing AI data usage. Confirming the candidate’s knowledge in current regulations ensures you are hiring someone aware of the legal landscape of AI.
Transparency in AI solutions
A transparent AI system is an epitome of trustworthiness, making the AI solution’s decision-making process understandable to stakeholders. Evaluating a candidate's approach to AI system transparency gives you an insight into their problem-solving ability in a dynamic environment.
Understanding of Responsible AI
Does the candidate grasp what Responsible AI entails? Do they understand the need to develop AI systems that are accountable, interpretive, robust and ethical? Their comprehension of Responsible AI, therefore, must be ascertained.
Handling stakeholders' concerns about an AI/ML model's trustworthiness
Stakeholders are key in any AI project. Their concerns, especially those related to the trustworthiness of AI systems, should be addressed satisfactorily. How a candidate deals with such issues provides a clue into their ability to manage concerns, and build AI systems of high integrity.
Importance of trust in AI systems
An AI system must be trustworthy. It is beneficial for the stakeholder and the general public. Getting a candidate's view on this issue helps you gauge their understanding of integrity in the AI environment.
Contributions to ethically sound AI projects
What has the candidate done to ensure AI projects are ethically sound? What roles have they played? This question will enable you to estimate their grasp of ethics in AI and see if they are a good fit for your team.
AI data privacy management
AI data privacy is a critical aspect of ethical AI. It would be prudent to ask the candidate to provide examples of how they have managed AI data privacy in past projects to assess their adeptness in this area.
Adjusting models or data sets for fairness
Ensuring the fairness of AI models is crucial. Therefore, asking the candidate about a time when they had to adjust a model or data set for fairness will give you a glimpse of their practical experience in dealing with biased AI systems.
Familiarity with open-source tools
Using open-source tools like TensorFlow, Pytorch, and Keras is common in AI project development. Thus, it’s crucial to establish the candidate's proficiency in using these tools.
Formal training in AI Ethics
Formal training in AI Ethics presents an added advantage. It shows a candidate's commitment to understanding the ethical and legal aspects of AI. Hence, asking about any AI ethics certifications or training helps gauge their level of dedication.
Creating robust AI systems
The aspiration to build robust AI systems while ensuring algorithmic fairness can be a tough balancing act. Therefore, a good understanding of a candidate's approach to this aspect goes a long way to assess their problem-solving aptitude.
Validating the robustness of an AI algorithm
Establishing the integrity of an AI algorithm is critical. A prospective candidate must have the ability to validate the robustness of the AI systems they develop.
Human oversight in AI decision-making
Despite the rise in automated decision-making systems, the role of human oversight remains indispensable. As such, understanding the candidate's view on this issue can give an insight into their willingness to incorporate humans in AI projects.
Experience with reinforcement learning models
Reinforcement learning models are critical components in developing intelligent and versatile AI systems. Checking whether the candidate has experience in implementing these models is necessary.
Designing for AI transparency
Seeking to understand the challenges a candidate might encounter while designing for AI transparency, and how they would overcome them can give a clue on their problem-solving skills and creativity.
Ethical considerations while handling AI data
The applicant should also have experience in handling AI data of various demographics with the due ethical considerations. This ensures varied data sets are used without discrimination, promoting inclusivity and fairness.
Keeping updated on ethical AI research and standards
AI is a rapidly evolving field with continuous research. Getting the perspective of the candidate on how they stay updated on ethical AI research and standards is essential.
Prescreening questions for Trustworthy AI Engineer
- Can you describe your experience with programming languages commonly used in AI like Python, Java, and R?
- What methodologies do you use to handle bias in machine learning models?
- Can you explain an instance where you suggested or implemented AI ethics and fairness in your projects?
- How familiar are you with the various regulations that guide AI data usage?
- What process do you follow to ensure transparency in the AI solutions you develop?
- Can you explain what Responsible AI means to you?
- Have you ever had to handle stakeholders' concerns about an AI/ML model's trustworthiness? How did you handle it?
- In your opinion, what are some important factors for trust in AI systems?
- Have you contributed to any AI project, where the primary aim was to ensure the outcomes were ethically sound?
- Can you provide examples of how you manage AI data privacy in your projects?
- Can you explain a time when you had to adjust a model or data set to be more fair or unbiased?
- How familiar are you with open-source tools like TensorFlow, Pytorch, and Keras?
- Do you have any certifications or formal training in AI Ethics?
- How do you approach creating a robust AI system while balancing it with algorithmic fairness?
- How do you validate the robustness of the AI algorithm you have developed?
- What is your view on human oversight in AI decision-making?
- Do you have any experience with implementing reinforcement learning models?
- What are some challenges one might face when designing for AI transparency and how would you overcome them?
- Can you describe ethical considerations involved while handling AI data of various demographics?
- How do you to stay updated on ethical AI research and standards?
Interview Trustworthy AI Engineer on Hirevire
Have a list of Trustworthy AI Engineer candidates? Hirevire has got you covered! Schedule interviews with qualified candidates right away.