Prescreening Questions to Ask Agricultural Data Scientist
When diving into the world of agricultural data science, it's crucial to ask the right questions to gauge a candidate’s expertise. In this article, we’ve compiled a comprehensive list of prescreening questions that can help illuminate a candidate’s experience, skills, and approach to various problems within this specialized field. Whether you’re an employer seeking to hire or someone prepping for an interview, these questions cover the essentials you need to focus on. Let's get started!
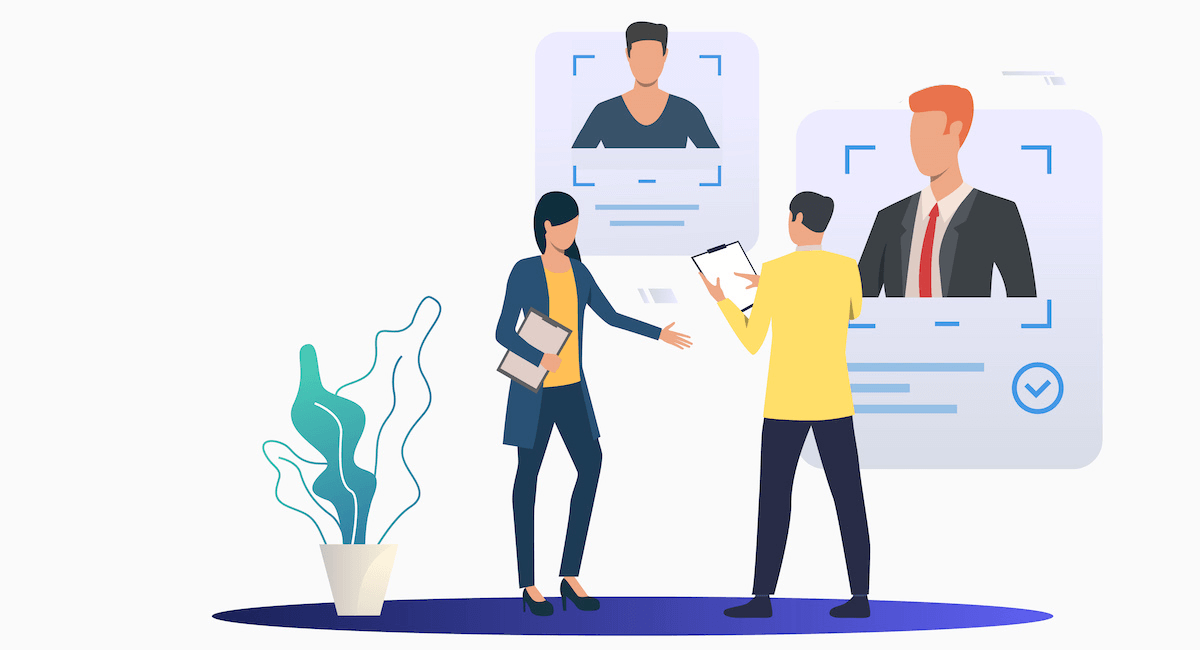
What experience do you have with agricultural datasets and what types of data have you worked with specifically?
Understanding a candidate’s familiarity with agricultural datasets is key. Have they worked with crop yield data, soil health metrics, or perhaps pesticide usage records? The variety of data types matters because it indicates their versatility and experience across different segments of agriculture.
How have you applied machine learning techniques to agricultural problems?
Machine learning is revolutionizing agriculture. So, ask them to describe specific instances where they've applied techniques like regression models, clustering, or supervised learning to tackle issues such as crop disease prediction or resource optimization.
Can you describe a project where you used remote sensing data in agriculture?
Remote sensing data, gathered from satellites or drones, can provide invaluable insights. Have they leveraged this data to monitor crop health, estimate yields, or manage water resources?
What tools and programming languages are you proficient in for data analysis?
From Python and R to specialized tools like QGIS and ArcGIS, the array of tools and programming languages they are proficient with can tell you a lot about their technical prowess.
Describe a time when your analysis directly impacted an agricultural decision.
This question gets to the heart of their real-world impact. Perhaps their data analysis led to a change in irrigation practices or influenced crop selection, demonstrating their practical value.
How do you stay current with advancements in agricultural data science?
The field of agricultural data science is rapidly evolving. Do they read journals, attend webinars, or maybe participate in online forums? Keeping up-to-date is crucial for staying relevant.
What experience do you have with geographic information systems (GIS) in agriculture?
GIS plays a significant role in modern agriculture. Their experience with GIS might include mapping soil types, planning pest management, or optimizing planting schedules.
Can you explain how you have used predictive analytics to benefit agricultural operations?
Predictive analytics can forecast yields, anticipate pest infestations, or predict soil conditions. Examples of their success in this area are particularly telling of their skill level.
Describe your approach to handling missing or incomplete agricultural data.
Data quality is paramount. How do they impute missing values or handle incomplete datasets? This reveals their methodological rigor and problem-solving abilities.
What statistical methods do you find most useful for analyzing agricultural data?
From t-tests and ANOVA to more sophisticated multivariate analyses, their preferred methods can provide insights into their analytical approach and depth of knowledge.
How do you validate the models you build for agricultural applications?
Model validation is essential to ensure accuracy and reliability. Do they use cross-validation, holdout datasets, or perhaps external validation datasets?
Explain a situation where you had to communicate complex data findings to non-technical stakeholders in the agriculture industry.
Communication skills are often as crucial as technical ones. How do they break down complex data insights into understandable terms for farmers, policymakers, or business managers?
Describe your experience with spatial data analysis in the context of agriculture.
Spatial data analysis can reveal patterns and correlations that aren't apparent in non-spatial data. Have they used it to study crop patterns, soil moisture levels, or pest infestations?
Have you ever worked on a project related to crop yield prediction or optimization? If so, please describe it.
Yield prediction is a cornerstone of agricultural data science. Past projects related to this topic can highlight their ability to handle one of the most critical aspects of farming.
What cloud platforms do you have experience with for storing and analyzing agricultural data?
Cloud platforms like AWS, Google Cloud, or Azure offer scalable solutions for data storage and analysis. Proficiency in these can be a significant asset.
How have you leveraged IoT devices for collecting agricultural data?
Internet of Things (IoT) devices are increasingly used to gather real-time data. Have they utilized sensors for soil moisture, weather stations, or even drones for crop monitoring?
Can you describe a project where you integrated weather data with agricultural data for analysis?
Integrating weather data can be a game-changer. This could involve combining weather forecasts with crop data to optimize planting times or manage irrigation.
What strategies do you use to ensure data quality and accuracy in your work with agricultural data?
Maintaining data quality is fundamental. Whether it's through regular data audits, using validation techniques, or employing data cleaning processes, their strategies provide a window into their commitment to precision.
Describe your experience with bioinformatics and its relevance to agriculture.
Bioinformatics might involve analyzing genetic data to improve crop strains or study pest genomes. Their experience here can indicate a higher level of expertise and interdisciplinary knowledge.
How do you approach the task of feature selection for models used in agricultural data science?
Feature selection can make or break a model. Do they use techniques like correlation analysis, stepwise selection, or perhaps more advanced methods like LASSO or Ridge Regression?
Prescreening questions for Agricultural Data Scientist
- What experience do you have with agricultural datasets and what types of data have you worked with specifically?
- How have you applied machine learning techniques to agricultural problems?
- Can you describe a project where you used remote sensing data in agriculture?
- What tools and programming languages are you proficient in for data analysis?
- Describe a time when your analysis directly impacted an agricultural decision.
- How do you stay current with advancements in agricultural data science?
- What experience do you have with geographic information systems (GIS) in agriculture?
- Can you explain how you have used predictive analytics to benefit agricultural operations?
- Describe your approach to handling missing or incomplete agricultural data.
- What statistical methods do you find most useful for analyzing agricultural data?
- How do you validate the models you build for agricultural applications?
- Explain a situation where you had to communicate complex data findings to non-technical stakeholders in the agriculture industry.
- Describe your experience with spatial data analysis in the context of agriculture.
- Have you ever worked on a project related to crop yield prediction or optimization? If so, please describe it.
- What cloud platforms do you have experience with for storing and analyzing agricultural data?
- How have you leveraged IoT devices for collecting agricultural data?
- Can you describe a project where you integrated weather data with agricultural data for analysis?
- What strategies do you use to ensure data quality and accuracy in your work with agricultural data?
- Describe your experience with bioinformatics and its relevance to agriculture.
- How do you approach the task of feature selection for models used in agricultural data science?
Interview Agricultural Data Scientist on Hirevire
Have a list of Agricultural Data Scientist candidates? Hirevire has got you covered! Schedule interviews with qualified candidates right away.