Prescreening Questions to Ask AI Algorithm Fairness Specialist
In today's world, where artificial intelligence (AI) is playing an increasingly significant role in our daily lives, ensuring fairness and avoiding biases in AI models are paramount. If you're looking to hire someone with expertise in AI fairness and bias detection, it's crucial to ask the right prescreening questions. Here's a comprehensive guide to the questions you should consider.
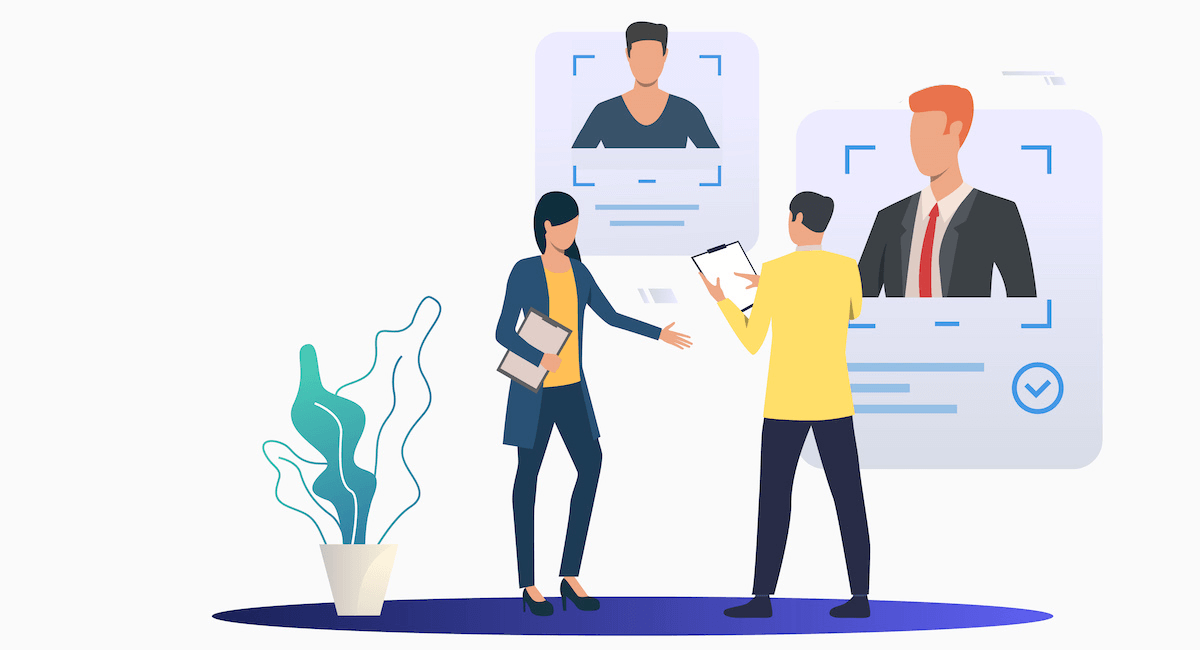
Describe your experience with bias detection and mitigation techniques in AI models.
When diving into the world of AI fairness, one of the first things you want to know is their hands-on experience. Have they worked on identifying biases in AI models? It's like unearthing hidden landmines that could cause unforeseen damage. Ask them to elaborate on specific techniques they've employed, such as fairness-aware machine learning, re-weighting, or even adversarial debiasing.
Can you explain the significance of disparate impact and how to measure it?
Disparate impact is a crucial concept in AI fairness. Imagine a situation where an AI model unintentionally discriminates against a particular group. How do we measure this? Understanding the candidate's grasp of metrics like the "80% rule" or how they use statistical parity difference can show their depth of knowledge on this topic.
How do you ensure the integrity of training data to prevent biases?
Garbage in, garbage out – that's the golden rule in AI. If the training data is biased, the model will be too. Ask them how they curate, clean, and verify the data. Maybe they use techniques like oversampling, undersampling, or even synthetic data generation to ensure the data's integrity.
What methods do you use to audit and monitor AI systems for fairness post-deployment?
Ensuring fairness isn't a one-and-done deal. It needs continuous monitoring. Do they set up fairness dashboards? Engage in regular audits? Understanding their post-deployment strategy can give you insights into their long-term commitment to AI fairness.
Describe a project where you successfully identified and addressed algorithmic bias.
Real-life examples can speak volumes. Ask them to recount a time they identified biases in a project. How did they address it? Did they re-engineer the model, retrain it with different data, or consult diverse groups for feedback? These insights can highlight their problem-solving skills.
How do you stay updated with ethical guidelines and regulations around AI fairness?
The AI fairness landscape is continuously evolving. Are they attending seminars, subscribing to relevant journals, or part of any professional communities? Their commitment to staying informed can be telling of their dedication to the cause.
What are some standard fairness metrics you consider when evaluating models?
There're a plethora of fairness metrics out there. From demographically parity to equalized odds, knowing which metrics they utilize can indicate their depth of knowledge. Do they opt for a one-size-fits-all approach, or are they adept at tailoring metrics to specific scenarios?
How do you approach fairness in AI when working with underrepresented groups in the data?
Underrepresented groups pose unique challenges. Do they use techniques like stratified sampling? Engage with community leaders to understand nuances? Their approach can reveal how empathetic and thorough they are when handling sensitive data.
Explain how you would handle a situation where a model’s fairness impacts its performance.
Balancing fairness and performance is like walking a tightrope. What do they prioritize? And how do they strike that balance? Their strategy can provide insights into their decision-making prowess and flexibility.
Describe your experience with explainable AI techniques to enhance algorithm transparency.
Transparency is key. Do they use techniques like LIME (Local Interpretable Model-agnostic Explanations), SHAP (SHapley Additive exPlanations), or others to make models interpretable? This ensures stakeholders understand how decisions are made, bolstering trust in the AI system.
Can you provide an example of how you’ve leveraged diverse teams to improve AI fairness?
Diverse perspectives can be gold mines for identifying hidden biases. Have they collaborated with teams from varied backgrounds? How did this collaboration enhance the fairness of the AI model?
What are the challenges in achieving fairness in AI algorithms and how do you address them?
Achieving fairness isn't a walk in the park. From insufficient data to entrenched societal biases, understanding the hurdles they've faced, and more importantly, how they overcame them, can be eye-opening.
Discuss how intersectionality can affect fairness assessments in AI algorithms.
Intersectionality complicates fairness assessments. How do they account for overlapping biases, like race and gender combined? Their approach to intersectionality can reveal their depth of understanding and innovative solutions.
How do you prioritize fairness features when designing AI systems?
Prioritization can be tricky. Do they have a checklist? Engage with stakeholders for feedback? Their methods can highlight their strategic planning and commitment to fairness.
What steps do you take to ensure stakeholder buy-in for fairness initiatives?
Stakeholder buy-in is crucial for any fairness initiative. How do they communicate the importance of fairness? Do they use metrics, real-life examples, or even simulations to get stakeholders on board?
Describe your experience with regulatory compliance related to fair algorithmic practices.
Regulations can make or break an AI project. Are they familiar with GDPR, CCPA, or other relevant regulations? Do they have a track record of ensuring their models comply with these standards?
How do you tackle the balance between algorithmic fairness and predictive accuracy?
Again, it's that tightrope. How do they ensure the model remains functional while being fair? Their strategies can provide insights into their balancing acts and depth of expertise.
What strategies do you use for continuous improvement of fairness in deployed AI models?
Once deployed, the work doesn't stop. Do they employ iterative testing, feedback loops, or even continual training? Their continuous improvement strategies can reveal their commitment to long-term fairness.
How do you educate and train team members on the importance of AI fairness?
Spreading the gospel of fairness is key. How do they onboard team members? Workshops? Regular training sessions? Their training methods can highlight their leadership qualities and commitment to a fair AI ecosystem.
Can you discuss a time when you had to convince leadership about the importance of a fairness-related change?
Convincing leadership can be a Herculean task. Do they have examples where they had to rally the troops for a fairness initiative? Their tales of these battles can shed light on their persuasive abilities and persistence.
Prescreening questions for AI Algorithm Fairness Specialist
- Describe your experience with bias detection and mitigation techniques in AI models.
- Can you explain the significance of disparate impact and how to measure it?
- How do you ensure the integrity of training data to prevent biases?
- What methods do you use to audit and monitor AI systems for fairness post-deployment?
- Describe a project where you successfully identified and addressed algorithmic bias.
- How do you stay updated with ethical guidelines and regulations around AI fairness?
- What are some standard fairness metrics you consider when evaluating models?
- How do you approach fairness in AI when working with underrepresented groups in the data?
- Explain how you would handle a situation where a model’s fairness impacts its performance.
- Describe your experience with explainable AI techniques to enhance algorithm transparency.
- Can you provide an example of how you’ve leveraged diverse teams to improve AI fairness?
- What are the challenges in achieving fairness in AI algorithms and how do you address them?
- Discuss how intersectionality can affect fairness assessments in AI algorithms.
- How do you prioritize fairness features when designing AI systems?
- What steps do you take to ensure stakeholder buy-in for fairness initiatives?
- Describe your experience with regulatory compliance related to fair algorithmic practices.
- How do you tackle the balance between algorithmic fairness and predictive accuracy?
- What strategies do you use for continuous improvement of fairness in deployed AI models?
- How do you educate and train team members on the importance of AI fairness?
- Can you discuss a time when you had to convince leadership about the importance of a fairness-related change?
Interview AI Algorithm Fairness Specialist on Hirevire
Have a list of AI Algorithm Fairness Specialist candidates? Hirevire has got you covered! Schedule interviews with qualified candidates right away.