Prescreening Questions to Ask AI Bias Mitigation Specialist
Prescreening for bias in AI models isn't just a technical task; it's an ethical journey. Everyone involved in creating or managing AI systems needs to get this right. In this article, we'll walk you through essential questions you should be asking to identify and mitigate bias in machine learning models. If you're ready to dive into the world of AI ethics, let's get started!
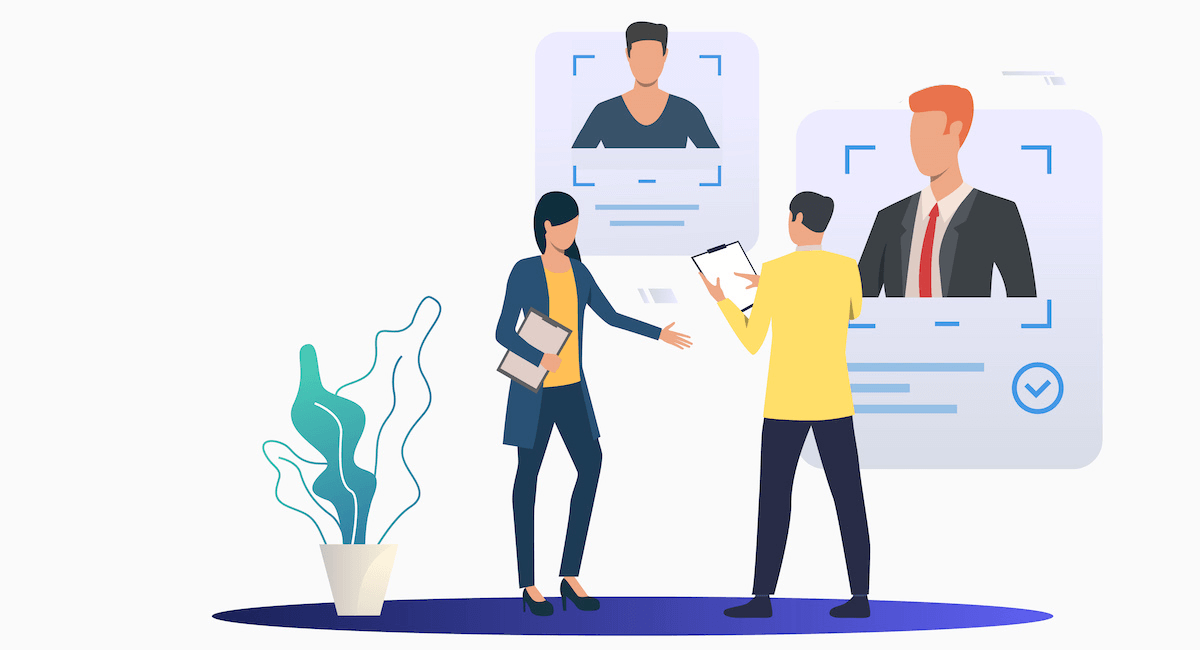
Can you describe your experience with identifying and mitigating bias in machine learning models?
Understanding someone's hands-on experience in tackling bias is the first step. Ask candidates to dig into their past projects. What roles did they play? Did they lead teams or contribute as a developer? Real-world examples help gauge their familiarity and expertise in the subject.
What tools or frameworks have you used for bias detection and mitigation?
Tools and frameworks like Fairlearn, AIF360, and TensorFlow's Model Analysis toolkit are buzzwords here. Knowing which ones they've used provides insight into their technical skill set and adaptability. Trust me, the right tools make a world of difference.
How would you handle a situation where you discover a critical bias in a high-stakes AI system?
This question is about problem-solving under pressure. How does one react to a crisis? A well-thought-out answer should include immediate steps to pause the model's deployment, a detailed investigation plan, and a long-term strategy for bias correction.
Can you give an example of a successful bias mitigation strategy you’ve implemented in the past?
Past success can be a good indicator of future performance. Look for specific examples where they identified bias, took corrective actions, and monitored the impact. It's like asking for a concrete victory story in the ongoing war against bias.
How do you stay current with the latest research and trends in AI ethics and bias mitigation?
The AI world moves fast. Keeping up with research papers, attending conferences, and participating in online forums are some ways professionals stay updated. It’s like staying on the cutting edge of a fast-evolving field.
What metrics do you use to evaluate bias in a dataset or model?
Metrics like Disparate Impact Ratio, Equal Opportunity Difference, and Demographic Parity Ratio are essential. By discussing these metrics, candidates demonstrate their ability to quantify and address bias, making them sound like data-savvy detectives.
Can you explain the difference between disparate impact and disparate treatment?
This is a textbook question. Disparate impact occurs when a model disproportionately affects a group based on race, gender, etc., though the criteria may be neutral. Disparate treatment, on the other hand, involves intentional bias. Understanding these differences is crucial for ethical AI development.
How would you approach the validation of an AI model to ensure it is free from harmful biases?
Validation is all about exhaustive testing and peer reviews. Candidates should talk about cross-validation, A/B testing, and fairness auditing to ensure the model performs well across all demographics. Think of it as the final dress rehearsal before the big show.
What role do fairness and transparency play in your approach to AI development?
Fairness ensures that every individual gets an equal shot, while transparency builds trust. Look for answers that show a commitment to open communication, thorough documentation, and ethical standards. These are the twin pillars holding up the integrity of AI projects.
How do you ensure that your bias mitigation strategies are both effective and sustainable?
Sustainability in bias mitigation involves continuous monitoring and updates. Ask them about their long-term plans, periodic audits, and how they keep the team educated on best practices. It's an ongoing marathon, not a one-time sprint.
What challenges have you faced in previous roles regarding bias mitigation, and how did you overcome them?
This question delves into troubleshooting expertise. Examples might include data imbalance, algorithmic opacity, or team resistance. The way they navigated these challenges offers a peek into their problem-solving mind.
How do you measure the success of a bias mitigation strategy?
Measurement could involve quantitative metrics and qualitative feedback. Success could be fewer flagged incidents in audits or increased user trust. Think of this as having a scorecard for your ethical report card.
What steps do you take to ensure that an AI model is fair to all demographic groups?
Steps like diversifying datasets, employing fairness metrics during model training, and conducting regular bias audits are crucial. A thoughtful approach here ensures no group is left behind, like an inclusive party where everyone gets an invitation.
Describe your experience working with diverse datasets and ensuring they are representative.
Experience with diverse datasets means dealing with data that mirrors real-world variety. It’s about knowing how to balance, augment, and validate datasets to ensure they're as inclusive as possible. Imagine it as a painter having a full palette to create an accurate picture.
How do you balance the need for model performance with the need for fairness?
Balancing performance and fairness is a classic dilemma. Look for strategies that include performance trade-offs, multi-objective optimization, and stakeholder consultations. It's like walking on a tightrope, weighing both sides equally.
How would you work with a team to educate them about bias and ethical considerations in AI?
Education involves workshops, regular training sessions, and open forums for discussion. Candidates might talk about creating easily digestible resources or mentoring team members. Picture it as planting seeds of knowledge for a future ethical AI forest.
What strategies would you use to audit a machine learning model for potential biases?
Strategies like algorithmic audits, peer reviews, and using specialized tools are key. Detailed audits reveal hidden biases, much like a thorough detective work uncovers concealed truths.
How do you handle trade-offs between different types of fairness (e.g., equal opportunity vs. demographic parity)?
Handling trade-offs involves understanding the implications of each type of fairness. It requires stakeholder inputs and balancing ethical considerations with practical constraints. It’s essentially a balancing act, like juggling multiple balls without dropping any.
Can you discuss a situation where biased data led to an unexpected outcome?
Real-world examples where biased data caused unforeseen issues offer valuable lessons. How they fixed it gives insights into their problem-solving skills. Think of it as a plot twist in a mystery novel that leads to an unexpected revelation.
What are some common pitfalls in bias mitigation and how can they be avoided?
Common pitfalls include over-reliance on biased historical data, ignoring intersectionality, and lack of continuous monitoring. Avoiding these requires vigilance, ongoing education, and robust frameworks. It's like navigating a minefield, where knowing the terrain can prevent missteps.
Prescreening questions for AI Bias Mitigation Specialist
- Can you describe your experience with identifying and mitigating bias in machine learning models?
- What tools or frameworks have you used for bias detection and mitigation?
- How would you handle a situation where you discover a critical bias in a high-stakes AI system?
- Can you give an example of a successful bias mitigation strategy you’ve implemented in the past?
- How do you stay current with the latest research and trends in AI ethics and bias mitigation?
- What metrics do you use to evaluate bias in a dataset or model?
- Can you explain the difference between disparate impact and disparate treatment?
- How would you approach the validation of an AI model to ensure it is free from harmful biases?
- What role do fairness and transparency play in your approach to AI development?
- How do you ensure that your bias mitigation strategies are both effective and sustainable?
- What challenges have you faced in previous roles regarding bias mitigation, and how did you overcome them?
- How do you measure the success of a bias mitigation strategy?
- What steps do you take to ensure that an AI model is fair to all demographic groups?
- Describe your experience working with diverse datasets and ensuring they are representative.
- How do you balance the need for model performance with the need for fairness?
- How would you work with a team to educate them about bias and ethical considerations in AI?
- What strategies would you use to audit a machine learning model for potential biases?
- How do you handle trade-offs between different types of fairness (e.g., equal opportunity vs. demographic parity)?
- Can you discuss a situation where biased data led to an unexpected outcome?
- What are some common pitfalls in bias mitigation and how can they be avoided?
Interview AI Bias Mitigation Specialist on Hirevire
Have a list of AI Bias Mitigation Specialist candidates? Hirevire has got you covered! Schedule interviews with qualified candidates right away.