Prescreening Questions to Ask Algorithmic Accountability Specialist
Ever wondered how algorithms can sometimes seem like mysterious black boxes, churning out results without explaining their reasoning? If so, you're not alone. The field of algorithmic accountability dives deep into these questions, striving to make algorithms fairer, more transparent, and ultimately, more trustworthy. In this article, we're focusing on a range of incisive questions that can help unpack someone's expertise and views on algorithmic accountability. Ready to dive in? Let's go!
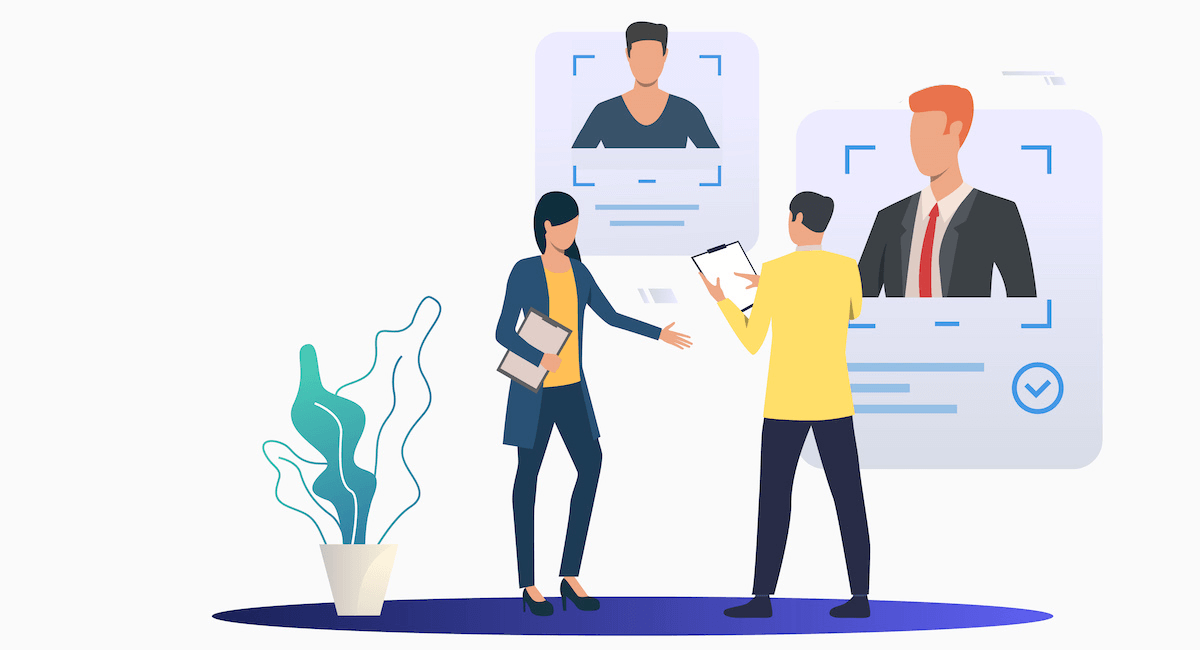
What inspired your interest in algorithmic accountability?
Understanding what sparked someone's interest can give you a glimpse into their passion for the field. Did they stumble upon a biased algorithm that piqued their curiosity, or was there a specific event or project that lit the fire within them? Everyone's story is unique, and these narratives often set the stage for what expertise they bring to the table.
How do you approach identifying potential biases in algorithms?
Investigating biases in algorithms is like being a detective. People might use various methods: analyzing data inputs, scrutinizing algorithmic outputs, or employing auditing tools. Each approach reflects a different aspect of tackling this complex issue. How rigorous are they? Do they rely on established methodologies or innovative techniques? It's all about the approach.
Describe your experience with algorithmic fairness assessment tools.
Knowing which tools someone has used can tell you a lot about their hands-on experience. Have they worked with well-known tools like Fairness Indicators or Aequitas, or have they developed custom solutions? The tools they use can speak volumes about their technical skills and familiarity with the latest tech in algorithmic fairness.
What methodologies do you use to measure the transparency of an algorithm?
Measuring transparency is critical for earning trust. Are they using explainable AI (XAI) techniques, or do they prefer model interpretability methods? Transparent methodologies can range from simple visualizations to complex mathematical models. Their chosen method reveals their depth of knowledge and their prioritization of clarity.
How would you explain the importance of transparency in machine learning?
Imagine trusting a chef who won't reveal the ingredients of their secret sauce. Transparency in machine learning is just as crucial—without it, how can users trust the results? Hearing how someone explains this vital concept can illuminate their ability to break down complex topics into digestible pieces for a broader audience.
Can you discuss a time when you identified and mitigated an unintended consequence of an algorithm?
Real-world examples are invaluable. Have they ever encountered an algorithm gone rogue? What steps did they take to address and rectify the issue? Stories like these provide practical insights into their problem-solving skills and their ability to mitigate risks proactively.
What are your views on the ethical implications of machine learning?
This question dives into their moral compass. Ethical dilemmas in machine learning are plentiful. Do they focus on issues of fairness, privacy, or social impact? Their views will shed light on how they navigate these thorny questions in their work.
Describe your experience with AI and machine learning model validation.
Validating models is like kicking the tires before buying a car. How do they ensure the models perform as expected? Whether through cross-validation, out-of-sample testing, or real-world trials, their approach to validation reveals their commitment to accuracy and reliability.
Have you ever worked with regulatory compliance in the context of AI algorithms?
Compliance isn't the most glamorous part of AI, but it's essential. Have they tangled with GDPR, CCPA, or other regulatory frameworks? Understanding their experience in this area can highlight their ability to navigate the legal landscape of algorithmic accountability.
How do you ensure that diverse perspectives are considered during algorithm development?
Diversity isn't just a buzzword—it's a necessity. How do they incorporate different viewpoints to avoid bias? Involving stakeholders from varied backgrounds, using diverse datasets, or implementing fairness audits can each play a role. Their strategy speaks to their inclusiveness and preventive measures against bias.
What metrics do you consider when evaluating an algorithm for fairness and bias?
From disparate impact to equal opportunity, many metrics can gauge fairness and bias. Which ones do they prioritize? Their chosen metrics can provide a window into how they quantify and strive for equity in their models.
How familiar are you with the latest research in algorithmic accountability and fairness?
Staying updated is like keeping your toolkit sharp. Are they avid readers of journals and papers, or active participants in conferences and forums? Their engagement with the latest research shows their dedication to staying at the forefront of the field.
What steps do you take to ensure the privacy and security of data used in algorithms?
Data privacy is paramount. Do they use encryption, anonymization, or access control mechanisms? Their practices for safeguarding data reflect their commitment to ethical standards and legal requirements.
Can you discuss a specific project where you applied principles of algorithmic accountability?
Spotlighting a particular project can illustrate their hands-on experience. What challenges did they face? What outcomes did they achieve? This kind of detailed example can showcase their practical skills and successes in applying theoretical principles.
How do you stay current with developments and best practices in this field?
Continuing education is vital in such a fast-evolving field. Do they attend workshops, enroll in online courses, or participate in professional communities? Their approach to learning demonstrates their commitment to maintaining and advancing their expertise.
What role do stakeholder and community feedback play in your accountability assessments?
Feedback is a critical component of accountability. Do they involve stakeholders early and often? How do they integrate community insights? Their methods for gathering and acting on feedback show their commitment to transparency and responsiveness.
What strategies do you employ to communicate complex algorithmic issues to a non-technical audience?
Translating technical jargon into understandable terms is like being a language interpreter. Do they use analogies, visual aids, or storytelling? Their communication strategies reveal their ability to bridge the gap between tech experts and everyday people.
What tools and frameworks do you prefer for audit and assessment of algorithms?
The tools and frameworks they prefer can tell you a lot about their technical preferences and expertise. Do they lean towards open-source tools, proprietary software, or custom-built solutions? Their choices can provide insights into their workflows and priorities.
How do you handle situations where there is resistance to implementing changes for accountability?
Resistance is inevitable in any field. How do they persuade stakeholders to embrace necessary changes? Do they rely on data-driven arguments, ethical appeals, or regulatory compliance? Their strategies for overcoming resistance reveal their leadership and negotiation skills.
What is your experience working in interdisciplinary teams to address algorithmic issues?
Complex problems often require diverse expertise. How do they collaborate with professionals from various fields, such as ethics, law, and domain-specific experts? Their experiences here show their ability to work effectively within broader teams for holistic solutions.
Prescreening questions for Algorithmic Accountability Specialist
- What inspired your interest in algorithmic accountability?
- How do you approach identifying potential biases in algorithms?
- Describe your experience with algorithmic fairness assessment tools.
- What methodologies do you use to measure the transparency of an algorithm?
- How would you explain the importance of transparency in machine learning?
- Can you discuss a time when you identified and mitigated an unintended consequence of an algorithm?
- What are your views on the ethical implications of machine learning?
- Describe your experience with AI and machine learning model validation.
- Have you ever worked with regulatory compliance in the context of AI algorithms?
- How do you ensure that diverse perspectives are considered during algorithm development?
- What metrics do you consider when evaluating an algorithm for fairness and bias?
- How familiar are you with the latest research in algorithmic accountability and fairness?
- What steps do you take to ensure the privacy and security of data used in algorithms?
- Can you discuss a specific project where you applied principles of algorithmic accountability?
- How do you stay current with developments and best practices in this field?
- What role do stakeholder and community feedback play in your accountability assessments?
- What strategies do you employ to communicate complex algorithmic issues to a non-technical audience?
- What tools and frameworks do you prefer for audit and assessment of algorithms?
- How do you handle situations where there is resistance to implementing changes for accountability?
- What is your experience working in interdisciplinary teams to address algorithmic issues?
Interview Algorithmic Accountability Specialist on Hirevire
Have a list of Algorithmic Accountability Specialist candidates? Hirevire has got you covered! Schedule interviews with qualified candidates right away.