Prescreening Questions to Ask Algorithmic Bias Auditor
Algorithmic bias is a critical issue in the world of AI and machine learning. It's like trying to make sure every slice of a pie is cut equally, no matter who’s at the table. If you're in the process of hiring someone to evaluate and mitigate these biases, asking the right prescreening questions is essential. Here, we'll cover some key questions to ask your candidates. Let’s dive right in!
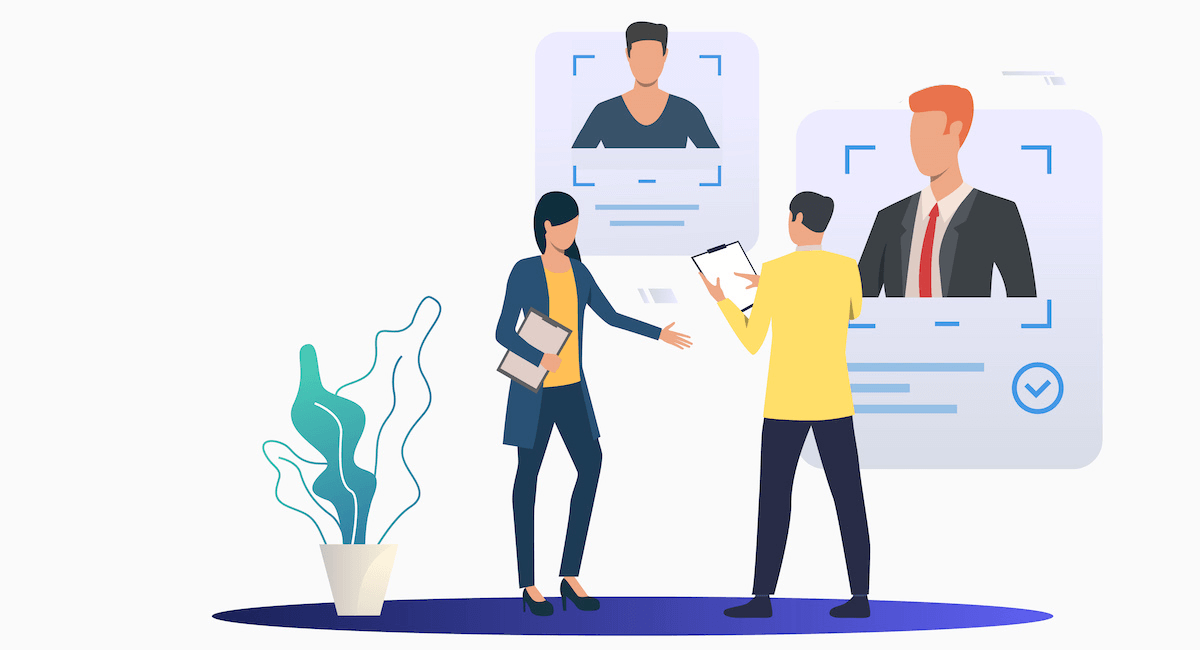
What experience do you have with identifying and addressing algorithmic bias?
Start with the basics. Experience speaks volumes. Does the candidate have hands-on experience dealing with algorithmic bias? Their answer will give you a glimpse into their background and how they’ve tackled similar challenges in the past. Experience is more than just knowledge; it's a testament to their ability to handle real-world problems.
Can you explain a situation where you successfully mitigated bias in a machine learning model?
Real-world examples are always fantastic. If they've tackled bias before, how did they do it? This question allows them to showcase their problem-solving skills and methodologies. Plus, it’s a great way to know if their approach aligns with your company’s standards and ethics.
What techniques do you use to detect bias in algorithms?
Techniques can vary from statistical analysis to more sophisticated methods like fairness-aware machine learning algorithms. Ask your candidate about the tools and techniques they employ. It’s like asking a chef about their favorite kitchen gadgets; their answers can tell you a lot about their proficiency.
How do you stay current with developments in fairness and ethics in AI?
AI and machine learning are fields that evolve rapidly. How does your candidate keep up? Are they attending conferences, reading academic papers, or following thought leaders on social media? Staying current is vital for tackling bias efficiently and effectively.
What data sources do you evaluate to check for bias in AI systems?
Data is the backbone of any AI system. What kind of data sources does your candidate evaluate to check for bias? Diverse sources ensure a well-rounded model, free from echo chambers that can propagate bias.
Have you worked with diverse datasets, and how do you ensure they are representative?
Working with diverse datasets is crucial. Ask them if they have experience with datasets that span different demographics and use cases. How do they ensure these datasets are representative of the real-world scenarios your AI model will encounter?
What tools or frameworks do you prefer for algorithmic bias auditing?
There are plenty of tools available for auditing bias, like AI Fairness 360 or Fairlearn. Which ones does your candidate prefer and why? Their choice can give you insights into their auditing process and their proficiency with different frameworks.
How do you approach the balance between model accuracy and fairness?
Ah, the age-old tug-of-war between accuracy and fairness. How does your candidate strike this balance? It’s like walking a tightrope; knowing when to prioritize one over the other is crucial for building ethical AI systems.
Can you discuss a time when you found resistance to addressing bias and how you handled it?
Change is often met with resistance. Has your candidate faced pushback when trying to address bias? How did they handle it? This question can reveal their leadership and conflict-resolution skills.
What metrics do you consider for evaluating the fairness of an algorithm?
Fairness isn't a one-size-fits-all kind of deal. What metrics does your candidate consider essential? Whether it's disparate impact ratio, equal opportunity difference, or another metric, their choice can tell you a lot about their evaluation process.
What steps do you take to validate that a model is free from bias before deployment?
Validation is vital before any model goes live. What steps does your candidate take to ensure the model is as bias-free as possible? This question digs into their pre-deployment workflow, which is crucial for safeguarding ethical standards.
How do you handle cases where bias is inherent in the training data?
Training data is often a culprit for hidden biases. How does your candidate deal with scenarios where the bias is baked into the data itself? Their approach can tell you if they are proactive in diagnosing and resolving such deep-rooted issues.
Can you explain a specific example where you identified hidden biases in an algorithm?
Specific examples provide concrete proof of their skills. Ask them to walk you through a case where they identified hidden biases. Their explanation can offer a window into their diagnostic process and attention to detail.
What are your views on the ethical implications of biased algorithms?
Ethics play a huge role in AI. What does your candidate think about the ethical implications of biased algorithms? Understanding their views can help you gauge if they align with your organization's ethical standards.
How do you prioritize which models to audit in a larger system?
When you have multiple models in a system, prioritization is critical. How does your candidate decide which models to audit first? Their priorities can reflect their strategic thinking and understanding of system-wide impacts.
What role does transparency play in your auditing process?
Transparency is often cited as a key to trust in AI. How transparent is your candidate in their auditing process? Their approach to transparency can reveal how they build trust and ensure accountability.
How would you incorporate stakeholder feedback into your bias auditing process?
Stakeholders are like the co-pilots of a project. How does your candidate incorporate their feedback? Their answer can tell you if they value diverse perspectives and utilize them to improve the auditing process.
What challenges do you foresee when auditing for algorithmic bias and how would you overcome them?
Every job has its challenges. What hurdles do they anticipate in auditing for algorithmic bias? More importantly, how do they plan to overcome them? This reveals their foresight and problem-solving capabilities.
Do you have experience with specific industries or applications where algorithmic bias is a significant concern?
Bias in healthcare AI is different from bias in financial algorithms. Does your candidate have experience in a specific industry? Their domain expertise can offer insights into their specialized skills.
How do you document and report findings related to algorithmic bias to non-technical stakeholders?
Communicating technical findings to non-technical stakeholders is an art. How does your candidate bridge this gap? Their communication skills are crucial, especially in multidisciplinary teams.
Prescreening questions for Algorithmic Bias Auditor
- What experience do you have with identifying and addressing algorithmic bias?
- Can you explain a situation where you successfully mitigated bias in a machine learning model?
- What techniques do you use to detect bias in algorithms?
- How do you stay current with developments in fairness and ethics in AI?
- What data sources do you evaluate to check for bias in AI systems?
- Have you worked with diverse datasets, and how do you ensure they are representative?
- What tools or frameworks do you prefer for algorithmic bias auditing?
- How do you approach the balance between model accuracy and fairness?
- Can you discuss a time when you found resistance to addressing bias and how you handled it?
- What metrics do you consider for evaluating the fairness of an algorithm?
- What steps do you take to validate that a model is free from bias before deployment?
- How do you handle cases where bias is inherent in the training data?
- Can you explain a specific example where you identified hidden biases in an algorithm?
- What are your views on the ethical implications of biased algorithms?
- How do you prioritize which models to audit in a larger system?
- What role does transparency play in your auditing process?
- How would you incorporate stakeholder feedback into your bias auditing process?
- What challenges do you foresee when auditing for algorithmic bias and how would you overcome them?
- Do you have experience with specific industries or applications where algorithmic bias is a significant concern?
- How do you document and report findings related to algorithmic bias to non-technical stakeholders?
Interview Algorithmic Bias Auditor on Hirevire
Have a list of Algorithmic Bias Auditor candidates? Hirevire has got you covered! Schedule interviews with qualified candidates right away.