Prescreening Questions to Ask Gene Expression Design Consultant
So, you're on the hunt for someone with a knack for gene expression analysis? That's awesome! But let’s face it, finding the right person who is both proficient and passionate in this niche can be a bit like finding a needle in a haystack. To help you out, I've put together some key prescreening questions you should ask. These will give you a better sense of who you're talking to and whether they have the chops for your project. Ready? Let’s dive in!
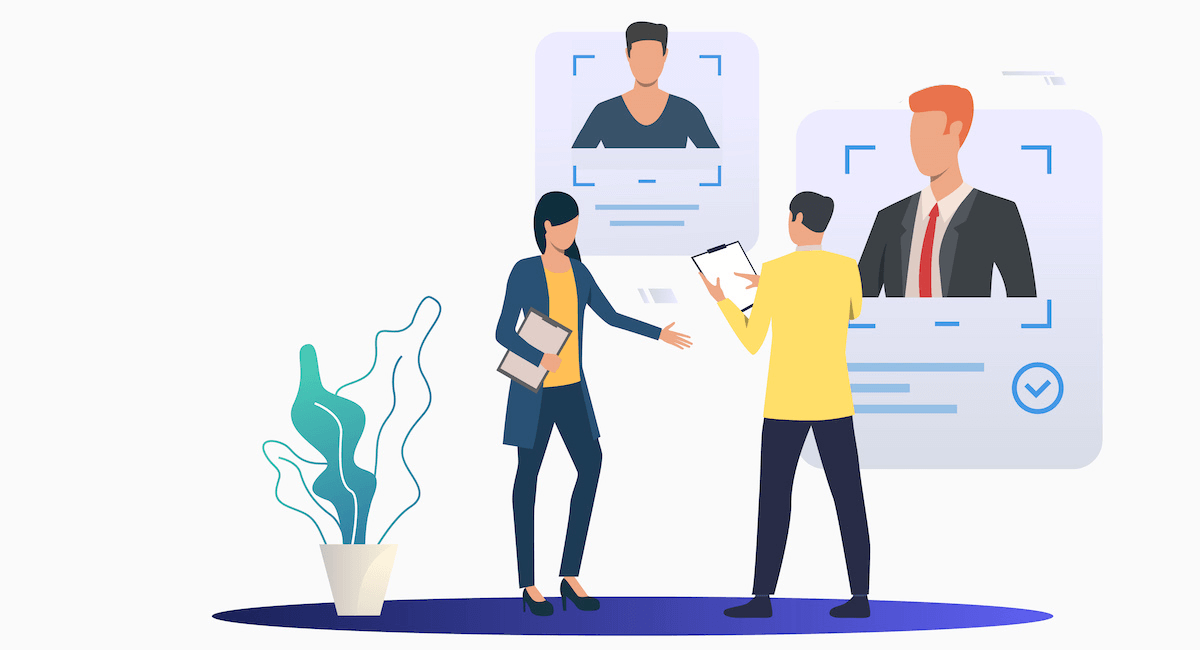
How do you stay current with advancements and technologies in gene expression analysis?
Staying current is super important because this field changes almost daily. I often ask candidates about their go-to resources. Do they read journals like Nature or Cell? Are they attending conferences or webinars? Some folks might even follow key influencers or experts in the field on social media. Having a continuous learning mindset is crucial for anyone in gene expression analysis.
Can you discuss your experience with different gene expression profiling techniques such as RNA-Seq, microarray, or qPCR?
This question helps you understand their hands-on experience. I usually expect answers that mention specific techniques like RNA-Seq for high-throughput sequencing or microarrays for a broader snapshot. Ask them to compare these techniques. Maybe, they prefer qPCR for its precision. The more detailed, the better!
What software and bioinformatics tools are you proficient in for analyzing gene expression data?
Tools are to gene expression analysts what brushes are to painters. Look for proficiency in tools like R, Bioconductor, or Python libraries like Pandas and NumPy. They might also mention specialized software like DESeq2 for differential expression analysis or Integrative Genomics Viewer (IGV) for data visualization. Diverse tool knowledge is a big plus.
Describe a challenging gene expression project you worked on and how you overcame the obstacles.
I love this question because it sheds light on their problem-solving skills. Real-life challenges can range from sample quality issues to computational bottlenecks. Listen for stories where they innovated solutions or pivoted strategies. It shows resilience and creativity, both of which are must-haves in this field.
How do you approach selecting the appropriate control samples for gene expression studies?
Controls can make or break an experiment. Good candidates will talk about matching controls as closely as possible to experimental samples — same tissue, same conditions, everything. They might also mention negative and positive controls to validate results. This ensures they understand the importance of minimizing variability.
What methods do you use to validate your gene expression results?
Validation is key. I want to hear about methods like technical replicates and independent validations using secondary techniques like qPCR. They might also discuss statistical methods to confirm the robustness of their findings. Trustworthy results are non-negotiable.
How do you ensure the reproducibility and accuracy of your gene expression experiments?
Reproducibility is a cornerstone of scientific integrity. Ideal answers will touch on using standardized protocols, maintaining meticulous lab notes, and employing rigorous statistical analyses. Consistency is key, and they should have concrete methods to ensure it.
Have you ever designed custom gene expression assays? If so, can you describe the process?
Designing custom assays requires a deep understanding of the subject. They should talk about selecting targets, optimizing primers, and validating the assay. It’s a sign of expertise if they can describe complex procedures clearly and concisely.
What is your experience with differential gene expression analysis?
Differential expression analysis helps identify which genes are up or down-regulated in different conditions. Look for experience with statistical methods like LIMMA or edgeR for this purpose. They should also be able to explain how they interpret these results in a biological context.
How do you handle large datasets from gene expression studies?
Big data can be overwhelming. They should be proficient in data handling and possibly cloud computing. Listen for mentions of using high-performance computing clusters or tools like Hadoop. Efficiency in managing vast datasets is crucial.
Can you discuss your experience with single-cell RNA sequencing?
Single-cell RNA-Seq is a game-changer. If they’ve worked with this, expect them to talk about the complexity of isolating single cells and the sophisticated algorithms needed for data analysis. Experience here indicates a high level of expertise.
What strategies do you use for normalizing gene expression data?
Normalization removes technical biases. They might talk about methods like TPM (Transcripts Per Million), FPKM (Fragments Per Kilobase of transcript per Million), or RPKM (Reads Per Kilobase of transcript, per Million mapped reads). Proper normalization ensures that results are comparable across samples and conditions.
How do you interpret gene expression data in the context of biological relevance?
Interpreting data is where the magic happens. They should mention pathways, gene ontology, and functional annotation tools like DAVID or KEGG. It's about connecting the dots between raw data and biological meaning.
Describe your experience with pathway analysis and gene ontology in gene expression studies.
Pathway analysis and gene ontology are like using a map to navigate a city. They help make sense of the data in a broader biological framework. Look for experience with tools like GSEA (Gene Set Enrichment Analysis) or Cytoscape for visualizing networks and pathways.
How do you tackle the problem of batch effects in gene expression data?
Batch effects are a common pitfall. Good candidates will mention statistical methods like ComBat or the use of batch effect correction algorithms. Handling this well ensures that results are not skewed by experimental artifacts.
What considerations do you take into account when designing a gene expression experiment?
Designing an experiment is all about planning. They should talk about sample sizes, randomization, replication, and ethical considerations. A well-designed experiment is the foundation of reliable results.
How do you handle missing or low-quality data in gene expression studies?
Data quality is everything. They might discuss methods for imputing missing values or discarding low-quality data. Balancing data integrity and usability is key, and how they handle this speaks volumes about their analytical rigor.
Describe your experience with integrative analysis involving gene expression and other omics data.
Integrative analysis is all about combining datasets for a more comprehensive view. They should mention techniques for multi-omics integration, like combining proteomics or metabolomics data with gene expression. This requires a broader skillset and indicates a more holistic approach to science.
How do you keep your gene expression analysis documentation organized and up to date?
Good documentation is like a clear roadmap. Look for answers mentioning digital lab notebooks, version control with tools like Git, and regular updates. Proper documentation ensures reproducibility and reliability.
Can you provide examples of how your work in gene expression design has impacted scientific research or clinical applications?
Impact stories are the best! Whether they've contributed to groundbreaking research or clinical diagnostics, real-world applications demonstrate the value of their work. This is the ultimate indicator of their expertise and relevance in the field.
Prescreening questions for Gene Expression Design Consultant
- What methods do you use to validate your gene expression results?
- What considerations do you take into account when designing a gene expression experiment?
- How do you stay current with advancements and technologies in gene expression analysis?
- Can you discuss your experience with different gene expression profiling techniques such as RNA-Seq, microarray, or qPCR?
- What software and bioinformatics tools are you proficient in for analyzing gene expression data?
- Describe a challenging gene expression project you worked on and how you overcame the obstacles.
- How do you approach selecting the appropriate control samples for gene expression studies?
- How do you ensure the reproducibility and accuracy of your gene expression experiments?
- Have you ever designed custom gene expression assays? If so, can you describe the process?
- What is your experience with differential gene expression analysis?
- How do you handle large datasets from gene expression studies?
- Can you discuss your experience with single-cell RNA sequencing?
- What strategies do you use for normalizing gene expression data?
- How do you interpret gene expression data in the context of biological relevance?
- Describe your experience with pathway analysis and gene ontology in gene expression studies.
- How do you tackle the problem of batch effects in gene expression data?
- How do you handle missing or low-quality data in gene expression studies?
- Describe your experience with integrative analysis involving gene expression and other omics data.
- How do you keep your gene expression analysis documentation organized and up to date?
- Can you provide examples of how your work in gene expression design has impacted scientific research or clinical applications?
Interview Gene Expression Design Consultant on Hirevire
Have a list of Gene Expression Design Consultant candidates? Hirevire has got you covered! Schedule interviews with qualified candidates right away.