Prescreening Questions to Ask Neuro-Symbolic AI for Scientific Discovery
Navigating the incredible world of neuro-symbolic AI is like trying to solve a massive jigsaw puzzle with pieces straight out of a scientific textbook. If you're in the process of finding the perfect candidate for a role that demands deep expertise in this area, these questions will help you zero in on the right fit. Whether it's integrating symbolic reasoning with deep learning or ensuring the scalability of AI systems, these inquiries will get you a peek into the mind of your potential hire. So, let’s dive into these must-ask questions and explore what they reveal about a candidate’s skills and experiences.
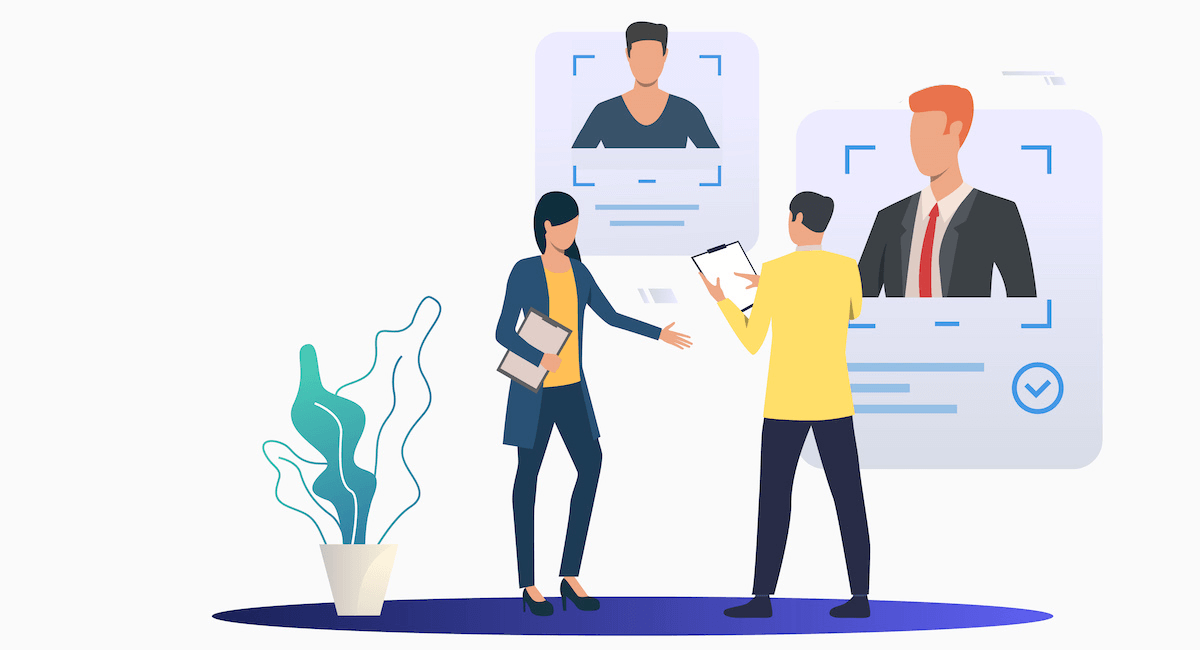
What is your experience with integrating symbolic reasoning and deep learning for scientific applications?
Here’s a great opener that's like asking someone to reveal their secret sauce. Symbolic reasoning and deep learning are like the peanut butter and jelly of AI—they work wonderfully together but require nuanced understanding. By asking about their experience, you’re probing into how well they can blend these complex components into a cohesive whole. Look for answers that highlight specific scientific applications, demonstrating not just theoretical knowledge but real-world execution.
Can you describe any projects where you have successfully implemented neuro-symbolic AI techniques?
This question is all about stories that showcase skills. You’re essentially asking the candidate to show their work, similar to how a magician reveals a trick but with the technical know-how. Successful implementation of neuro-symbolic AI isn’t just about know-how; it’s also about the how-to. Detailed stories about past projects give you insight into their problem-solving abilities and innovative thinking.
How do you approach the challenge of representing complex scientific knowledge in a machine-readable format?
Think of this as asking how someone translates a foreign language. Scientific knowledge is often complex and dense, much like an intricate tapestry. Making it machine-readable is a challenging task akin to weaving that tapestry into a digital format. Listen for strategies involving structured data, ontologies, and semantic layers. An effective approach indicates a deep understanding of both science and AI methodologies.
What strategies do you employ to ensure the interpretability of your AI models?
An unexplainable AI model can be a black box that no one wants to deal with. Think of this question as asking how they shine a light into that box. Interpretability is crucial, especially in scientific contexts where every decision needs to be justifiable. Look for answers that emphasize transparency and user-friendly design, which show they prioritize making the AI understandable to human users.
How do you handle incomplete or uncertain data in your neuro-symbolic AI models?
Data is rarely perfect; it’s often like Swiss cheese—full of holes. Handling incomplete or uncertain data requires a nuanced approach. The candidate should talk about imputation techniques, probabilistic models, or uncertainty quantification methods. Effective handling of such data indicates a robust yet flexible AI model design.
Can you discuss your experience with knowledge graphs and their use in scientific discovery?
Knowledge graphs are like the treasure maps of data science. They help in navigating the complex relationships between data points. By diving into this question, you're asking for a tour of their treasure-hunting expeditions. Concrete examples of how they've used knowledge graphs in scientific discovery will speak volumes about their understanding and innovation abilities.
What role do ontologies play in your approach to neuro-symbolic AI?
Ontologies are like the blueprints of structured knowledge. This question reveals how well they can draw and employ these blueprints in AI development. You’re looking for an explanation of how they use ontologies to organize and navigate complex scientific information and how this integrates with AI models.
How do you ensure the scalability of your neuro-symbolic AI systems for large datasets?
Scalability is the ultimate stress test. Imagine your AI system as a balloon; scalability ensures it expands without bursting. Answers should include technical strategies like distributed computing, cloud services, and data partitioning. A scalable solution is a strong indicator of a forward-thinking, practical approach to AI.
What techniques do you use to integrate domain-specific knowledge into neural networks?
Integrating domain-specific knowledge is like adding a secret ingredient to a family recipe. It can make all the difference. Look for specific techniques such as embedding approaches, transfer learning, or specialized training data. The focus here is on how personalized and finely-tuned their AI models can get.
Can you provide examples of how your neuro-symbolic AI solutions have accelerated scientific discovery?
Here, you're playing detective, seeking real-world evidence of their claims. Examples should ideally be grounded in tangible outcomes—research published, discoveries made, or significant time saved. This question separates the talkers from the doers, giving you a glimpse into the actual impact of their work.
How do you validate the accuracy and reliability of your AI models in scientific contexts?
Validation processes are like the quality control procedures in a factory, ensuring every product meets high standards. Expect detailed methodologies involving testing datasets, cross-validation, peer reviews, and possibly real-world trials. Strong validation methods indicate reliability and robustness of their AI models.
What is your approach to combining natural language processing with symbolic reasoning for scientific text analysis?
Combining NLP with symbolic reasoning is like translating ancient manuscripts while keeping their essence intact. Look for techniques that involve semantic parsing, entity recognition, and synthesis of symbolic and statistical methods. This reveals their ability to merge different AI approaches effectively.
How do you address the challenge of evolving scientific knowledge in your neuro-symbolic AI models?
Scientific knowledge is always evolving; it’s like a river flowing endlessly. How do they keep their AI models up-to-date? Listen for mechanisms like continuous learning, knowledge base updates, and dynamic model adjustments. Staying current in such a fast-paced environment is crucial for long-term success.
What methods do you use to facilitate the transfer of knowledge from one domain to another in your AI systems?
Knowledge transfer between domains is like being bilingual; it opens up new possibilities. Expect to hear about transfer learning, domain adaptation, and multi-task learning. These methods are key to creating versatile AI systems that can tackle challenges across different scientific fields.
How do you incorporate expert feedback into your neuro-symbolic AI models?
Expert feedback is gold—it's like adding final touches to a masterpiece. Inquire how they loop in this valuable input effectively. Look for mechanisms that integrate feedback to refine models iteratively, showcasing a dynamic and collaborative approach to AI development.
Can you describe your experience with causal reasoning in scientific AI applications?
Causal reasoning is the Sherlock Holmes of AI—it digs deep to find the 'why' behind the phenomena. This question unearths their skills in identifying causal relationships within data. Examples of causal modeling or intervention analysis will reveal their depth of understanding and application.
What strategies do you have for integrating multi-modal data sources in neuro-symbolic systems?
Multi-modal data integration is like juggling multiple balls; it requires skill and coordination. Ask them how they combine structured, unstructured, and semi-structured data. Their strategies for integration can indicate their ability to manage diverse data types for richer insights.
How do you ensure that your neuro-symbolic AI solutions are ethically sound and unbiased?
Ethics and bias in AI are critical—think of it as being a responsible wizard. This question probes their ethical considerations, data fairness procedures, and mitigation of biases. Look for comprehensive strategies that extend beyond standard protocols, aiming for genuinely unbiased and fair AI models.
What are your thoughts on the future directions of neuro-symbolic AI in scientific research?
Asking this is like looking into a crystal ball. This question reveals their vision and forward-thinking capabilities. Are they keeping an eye on emerging trends? Do they foresee advancements and potential applications? Clear and insightful thoughts indicate a candidate who’s not just skilled but also tuned into the future of neuro-symbolic AI.
How do you prioritize and manage trade-offs between accuracy, interpretability, and computational efficiency in your AI models?
Trade-offs in AI are inevitable, like balancing on a tightrope. This question examines their decision-making process when faced with these dilemmas. Insightful prioritization strategies reflect a deep understanding of the nuanced balance required to create effective, efficient, and interpretable AI models.
Prescreening questions for Neuro-Symbolic AI for Scientific Discovery
- What is your experience with integrating symbolic reasoning and deep learning for scientific applications?
- Can you describe any projects where you have successfully implemented neuro-symbolic AI techniques?
- How do you approach the challenge of representing complex scientific knowledge in a machine-readable format?
- What strategies do you employ to ensure the interpretability of your AI models?
- How do you handle incomplete or uncertain data in your neuro-symbolic AI models?
- Can you discuss your experience with knowledge graphs and their use in scientific discovery?
- What role do ontologies play in your approach to neuro-symbolic AI?
- How do you ensure the scalability of your neuro-symbolic AI systems for large datasets?
- What techniques do you use to integrate domain-specific knowledge into neural networks?
- Can you provide examples of how your neuro-symbolic AI solutions have accelerated scientific discovery?
- How do you validate the accuracy and reliability of your AI models in scientific contexts?
- What is your approach to combining natural language processing with symbolic reasoning for scientific text analysis?
- How do you address the challenge of evolving scientific knowledge in your neuro-symbolic AI models?
- What methods do you use to facilitate the transfer of knowledge from one domain to another in your AI systems?
- How do you incorporate expert feedback into your neuro-symbolic AI models?
- Can you describe your experience with causal reasoning in scientific AI applications?
- What strategies do you have for integrating multi-modal data sources in neuro-symbolic systems?
- How do you ensure that your neuro-symbolic AI solutions are ethically sound and unbiased?
- What are your thoughts on the future directions of neuro-symbolic AI in scientific research?
- How do you prioritize and manage trade-offs between accuracy, interpretability, and computational efficiency in your AI models?
Interview Neuro-Symbolic AI for Scientific Discovery on Hirevire
Have a list of Neuro-Symbolic AI for Scientific Discovery candidates? Hirevire has got you covered! Schedule interviews with qualified candidates right away.