Prescreening Questions to Ask AI Bias Mitigation Consultant
AI bias is a hot topic, and for a good reason. You want to ensure that your AI systems are not only effective but also ethical and fair. If you're on the lookout for an expert in AI bias detection and remediation, it's crucial to ask the right questions during prescreening. The questions below will help you gauge the candidate's experience, skills, and methodologies in handling AI bias.
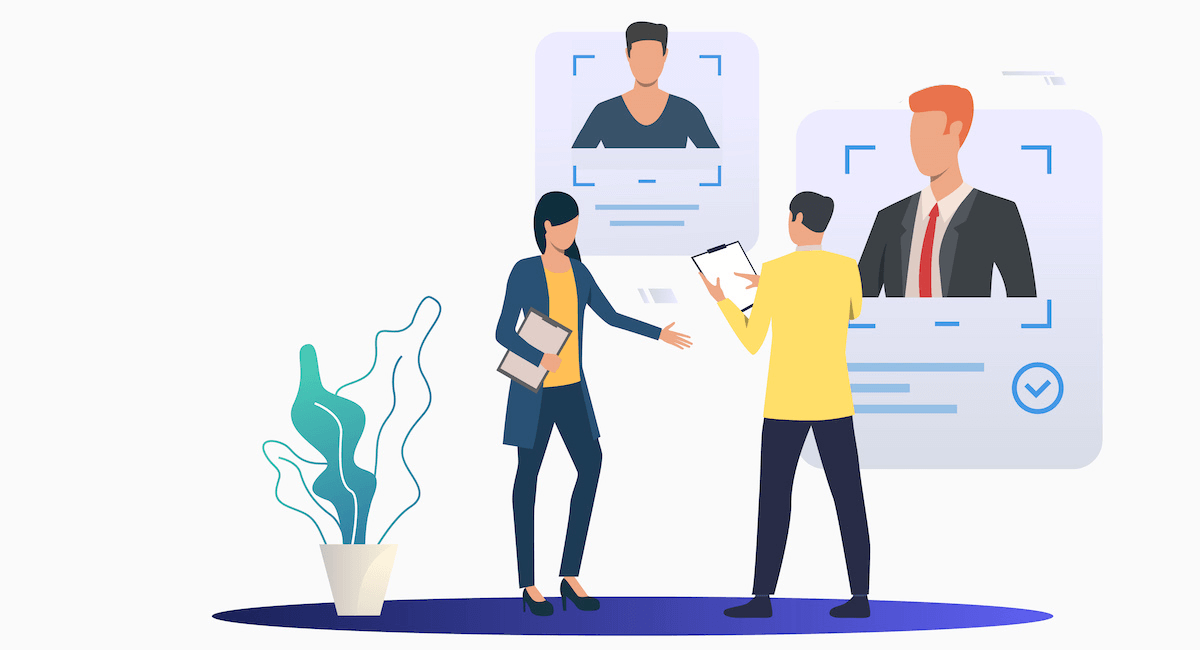
Describe your experience with AI bias detection and remediation.
When it comes to AI bias detection and remediation, experience counts. You'll want to gauge how seasoned the candidate is by asking them to describe their experience. Have they tackled similar projects before? What's their track record? Diving into specifics can help you understand their depth of knowledge and practical experience in the field.
Can you explain some successful AI bias mitigation projects you have previously worked on?
Success stories can be extremely telling about a candidate's capabilities. Ask them to recount specific projects where they've successfully mitigated AI bias. What was the project about? What challenges did they face, and how did they overcome them? These narratives can provide insight into their problem-solving skills and ingenuity.
What methodologies and tools do you use for identifying AI bias?
There are numerous methodologies and tools out there to identify AI bias, from statistical tests to specialized software. Ask the candidate about their go-to methods and tools. Are they using the latest technology? Are they versatile in adapting different methodologies based on the project needs? This will help you understand their technical proficiency.
How do you stay updated with the latest research and best practices in AI ethics and bias?
The world of AI ethics and bias is ever-evolving. You'll want someone who keeps up with the latest research and best practices. Ask them how they stay in the loop—do they attend conferences, partake in online courses, or follow specific journals or blogs? This will show their commitment to staying informed and up-to-date.
Explain your understanding of fairness definitions in the context of AI.
Fairness in AI can mean different things depending on the context. It's crucial to understand how the candidate defines fairness. Do they focus on fairness in terms of equal accuracy across groups, or do they prioritize minimizing disparate impacts? Their understanding of fairness will give you a good sense of their ethical framework and priorities.
How do you approach bias mitigation in datasets before model training?
Bias often starts in the data. Ask the candidate how they mitigate bias in datasets before even starting model training. Do they perform data cleaning, rebalancing, or augmentation? What strategies do they employ, and how effective have they been? This question can uncover their proactive measures in bias prevention.
What are some common sources of bias in AI systems you've encountered, and how did you address them?
AI systems can be biased in various ways, from data collection to algorithmic design. Ask for some common sources of bias they've encountered and how they tackled them. Did they identify biases in training data, feature selection, or even model deployment? Their response will give you insights into their analytical and problem-solving skills.
Can you discuss how you measure the effectiveness of bias mitigation strategies?
It's one thing to implement bias mitigation strategies; it's another to measure their effectiveness. How does the candidate assess whether their interventions are working? Do they use specific metrics or benchmarks? Understanding their evaluation process will help you gauge their thoroughness and analytical skills.
Describe your experience with regulatory compliance and ethical guidelines in AI development.
Regulatory compliance and ethical guidelines are increasingly important in AI development. Ask the candidate about their experience in this area. Are they familiar with GDPR, CCPA, or other regulations? How do they ensure their projects comply with these guidelines? Their response will reflect their understanding of legal and ethical considerations in AI.
How do you collaborate with data scientists and engineers to ensure the integration of bias mitigation strategies?
AI bias mitigation is often a team effort. Find out how the candidate collaborates with data scientists and engineers. Do they run workshops, share best practices, or work closely during model development? Their collaboration skills can significantly impact the effectiveness of bias mitigation strategies.
What strategies do you use to mitigate bias during the AI model validation and testing phases?
Bias can creep in at any stage, including validation and testing. Ask the candidate about their strategies for bias mitigation during these phases. Do they conduct bias audits, use fairness constraints, or deploy other techniques? This question can reveal their thoroughness and technical acumen.
Can you give examples of how you have handled resistance or challenges from stakeholders regarding bias mitigation measures?
Implementing bias mitigation strategies often involves overcoming resistance from stakeholders. Ask the candidate for examples of how they've handled such challenges. Did they use persuasion, compromise, or education to bring stakeholders on board? Their answer can reveal their people-management skills and perseverance.
How do you work with diverse teams to ensure a comprehensive approach to identifying and mitigating AI bias?
A diverse team can provide a more rounded perspective on bias. Ask how the candidate works with diverse teams to tackle AI bias. Do they actively seek input from team members with different backgrounds and expertise? Their collaborative approach can enhance the comprehensiveness of bias mitigation efforts.
What role do explainability and transparency play in your approach to AI bias mitigation?
Explainability and transparency are crucial for trust in AI systems. Ask the candidate about their approach to these aspects. Do they use interpretable models, maintain transparent processes, or provide clear documentation? Their commitment to explainability and transparency can significantly impact the success of bias mitigation.
How do you balance accuracy and fairness when mitigating bias in AI models?
Balancing accuracy and fairness is often a tricky task. How does the candidate navigate this challenge? Do they prioritize one over the other, or aim for an optimal balance? Their approach to this balancing act can reveal their decision-making process and ethical priorities.
Describe a time when you had to retrain or redevelop an AI model due to bias issues. What was the outcome?
Sometimes, despite best efforts, an AI model may need retraining due to bias issues. Ask the candidate to describe a time they faced this scenario. What led to the decision to retrain? What was the outcome? Their experience with such situations can shed light on their problem-solving skills and resilience.
What techniques do you recommend for continuous monitoring and updating of AI systems to prevent bias?
Continuous monitoring is essential to prevent bias from creeping back into AI systems. What techniques does the candidate recommend? Do they use automated tools, periodic audits, or regular updates? Their strategies for ongoing vigilance can help maintain the integrity and fairness of AI systems.
Can you discuss how intersectionality is considered in your bias mitigation strategies?
Intersectionality—considering multiple dimensions of identity—can complicate bias mitigation. Ask the candidate how they incorporate intersectionality into their strategies. Do they use specialized methods to identify intersecting biases? Their approach to intersectionality can reveal their thoroughness and inclusivity.
How do you handle situations where bias mitigation impacts model performance negatively?
Sometimes, bias mitigation can negatively impact model performance. Ask the candidate how they handle such situations. Do they explore alternative methods, seek to understand the trade-offs, or engage stakeholders in discussions? Their approach can indicate their problem-solving skills and resilience.
What frameworks or guidelines do you follow to ensure that your bias mitigation strategies are ethically sound?
Finally, ask the candidate about the frameworks or guidelines they follow to ensure their bias mitigation strategies are ethically sound. Are they guided by IEEE standards, AI ethics guidelines, or internal policies? Their adherence to recognized frameworks can give you confidence in their ethical commitment.
Prescreening questions for AI Bias Mitigation Consultant
- Describe your experience with AI bias detection and remediation.
- Can you explain some successful AI bias mitigation projects you have previously worked on?
- What methodologies and tools do you use for identifying AI bias?
- How do you stay updated with the latest research and best practices in AI ethics and bias?
- Explain your understanding of fairness definitions in the context of AI.
- How do you approach bias mitigation in datasets before model training?
- What are some common sources of bias in AI systems you've encountered, and how did you address them?
- Can you discuss how you measure the effectiveness of bias mitigation strategies?
- Describe your experience with regulatory compliance and ethical guidelines in AI development.
- How do you collaborate with data scientists and engineers to ensure the integration of bias mitigation strategies?
- What strategies do you use to mitigate bias during the AI model validation and testing phases?
- Can you give examples of how you have handled resistance or challenges from stakeholders regarding bias mitigation measures?
- How do you work with diverse teams to ensure a comprehensive approach to identifying and mitigating AI bias?
- What role do explainability and transparency play in your approach to AI bias mitigation?
- How do you balance accuracy and fairness when mitigating bias in AI models?
- Describe a time when you had to retrain or redevelop an AI model due to bias issues. What was the outcome?
- What techniques do you recommend for continuous monitoring and updating of AI systems to prevent bias?
- Can you discuss how intersectionality is considered in your bias mitigation strategies?
- How do you handle situations where bias mitigation impacts model performance negatively?
- What frameworks or guidelines do you follow to ensure that your bias mitigation strategies are ethically sound?
Interview AI Bias Mitigation Consultant on Hirevire
Have a list of AI Bias Mitigation Consultant candidates? Hirevire has got you covered! Schedule interviews with qualified candidates right away.