Prescreening Questions to Ask Neuro-Symbolic AI for Legal Reasoning
If you're delving into the world of neuro-symbolic AI, particularly in legal applications, you've struck gold! The amalgamation of symbolic reasoning and machine learning offers transformative possibilities in the legal realm, but it's crucial to ask the right questions before diving headfirst. This article narrows down significant prescreening questions that will provide a thorough understanding of integrating these advanced AI methods for legal tasks. These questions are key to discerning the depth of an individual's experience and the practical application of neuro-symbolic AI systems in the legal landscape.
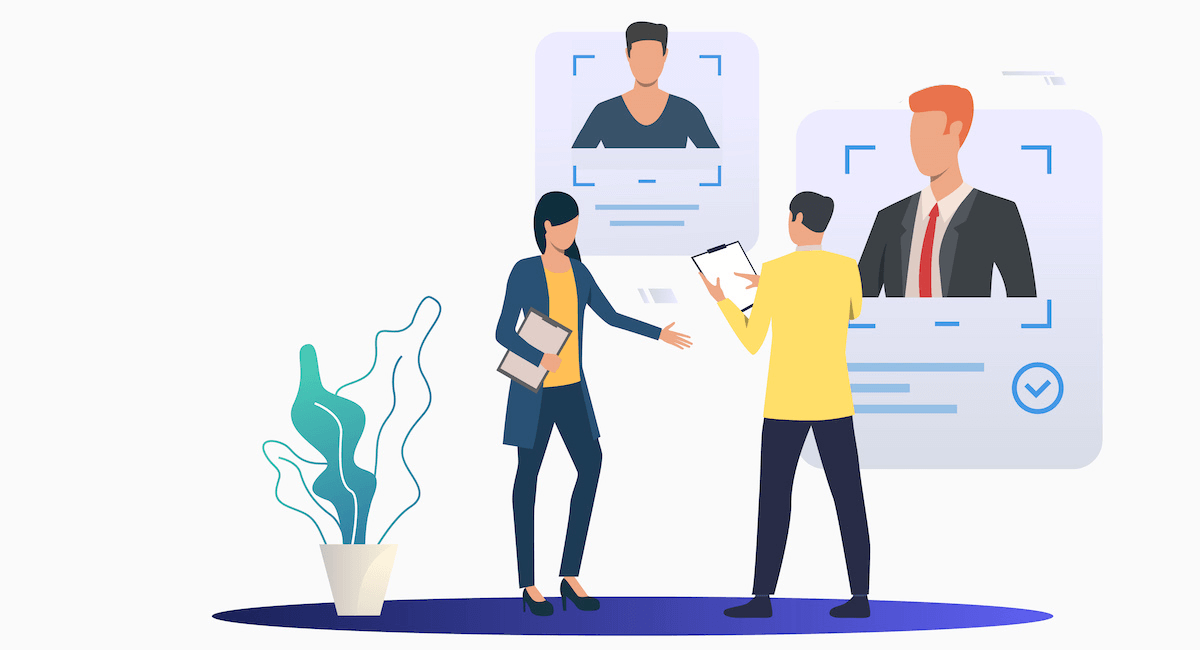
Can you describe your experience with integrating symbolic reasoning and machine learning techniques?
Let's kick things off by getting a sense of the basics. Integrating symbolic reasoning with machine learning isn’t everyone's cup of tea. So, hearing about one's hands-on experience can shed light on their skill level and past projects. Have they tackled challenges that needed a blend of logic-based and data-driven approaches? Their journey could provide insights into their versatility and problem-solving capabilities in this unique combination.
What are the key challenges you’ve encountered in developing neuro-symbolic AI systems for legal applications?
Developing neuro-symbolic AI for legal applications is no walk in the park. Understanding the hurdles faced gives a realistic picture of what you're up against. Are the challenges technical, ethical, or maybe even regulatory? This can tell you not just about the obstacles but also about the resilience and troubleshooting prowess of the person.
How do you ensure the accuracy and reliability of the AI’s legal reasoning?
Accuracy is the Holy Grail in AI, especially in legal matters where stakes are as high as skyscrapers. How does one guarantee that the AI’s reasoning is spot-on? Knowing the strategies for validation and testing can assure you that the person takes their task seriously, ensuring the outcomes are precise and reliable.
Can you explain how you handle ambiguous or conflicting legal information in your system?
Law is often grey rather than black and white. Ambiguities and contradictions are part and parcel of legal texts. The ability to handle these complexities effectively can make or break an AI system. How does the individual navigate through such murky waters? Their method can reveal their thought process and adaptability to real-world complexities.
What methods do you use to extract and represent legal knowledge for neuro-symbolic AI?
Extracting and representing legal knowledge is akin to sculpting a statue from a block of marble—meticulous and precise. The techniques used here can greatly affect the efficiency and performance of the AI system. Learning about their approach can highlight their expertise in managing legal data.
How do you evaluate the performance of your neuro-symbolic AI system in legal reasoning tasks?
Evaluation is crucial. It's like the report card of an AI system. Various metrics and benchmarks are used to gauge performance. This question helps understand how thorough and rigorous the evaluation process is and the reliability of the AI’s outcomes.
What kind of legal datasets have you used for training and testing your models?
Not all data is created equal. The quality and nature of legal datasets used for training and testing can spell the difference between success and failure. Knowing the types of datasets involved provides insights into the system's robustness and versatility.
Can you describe a successful implementation of a neuro-symbolic AI system for a legal domain?
Success stories are always inspiring and illuminating. Hearing about a project where everything clicked can give you concrete examples of the system’s potential. It’s a testament to the person's ability to apply theory in practice.
How does your system handle updates to laws and regulations over time?
Laws are living documents—they change. An AI system's ability to stay up-to-date is crucial. Does the system have a mechanism for integrating new regulations seamlessly? This reveals its adaptability and future-readiness.
What techniques do you use to integrate case law and statutory law in your reasoning process?
Case law and statutory law are like two sides of the same coin in legal reasoning. Blending these effectively within an AI system can be intricate. Unveiling these techniques can show the comprehensiveness and depth of the AI’s legal acumen.
How do you address explainability and transparency in your neuro-symbolic AI system?
In legal contexts, transparency isn’t just a buzzword—it’s a necessity. How does one make the AI’s decisions transparent and explainable? This question helps you gauge the system’s trustworthiness and the developer’s commitment to demystifying AI reasoning.
In what ways does your AI system support decision-making for legal professionals?
An AI system should serve as a trusty sidekick to legal professionals, aiding in decision-making. How exactly does it do this? Does it offer insights, predictions, or recommendations? This points to the practical applications and benefits of the system.
What approaches do you use to ensure compliance with ethical and legal standards in AI development?
Ethics and legal compliance are the twin pillars of trustworthy AI. Ensuring these involves a conscientious effort. What measures are taken to align with ethical standards? This sheds light on the integrity and responsibility ingrained in the development process.
Can your system identify and correct biases within legal data and reasoning processes?
Bias is an ever-present menace in AI. Recognizing and rectifying it is crucial, especially in legal reasoning. How does one tackle this? This reveals the robustness of bias mitigation strategies and commitment to fair outcomes.
How does your neuro-symbolic AI system manage the balance between logic-based and data-driven approaches?
Balancing logic-based reasoning with data-driven insights is like walking a tightrope. How is this equilibrium maintained? This question uncovers the nuanced approach to leveraging the strengths of both paradigms.
Can you discuss any collaborative projects with legal experts or institutions in developing your AI system?
Collaboration with legal experts enriches AI development. Such partnerships signal the integration of domain expertise with technical innovation. Have they worked with legal institutions? This can offer confidence in the system’s real-world applicability and acceptance.
How scalable is your neuro-symbolic AI system for different legal jurisdictions and areas of law?
Scalability ensures that an AI system isn't boxed into a corner. Can it adapt to various legal jurisdictions or expand into other areas of law? This indicates the system’s flexibility and potential for broader application.
What are some of the limitations of your current neuro-symbolic AI system for legal reasoning?
No system is perfect. Acknowledging limitations showcases honesty and a willingness to improve. What shortcomings exist, and what’s being done about them? This openness can actually build trust and set realistic expectations.
How do you ensure that your system maintains confidentiality and data privacy in handling legal data?
Legal data is sensitive, demanding stringent confidentiality and privacy measures. How is this information protected? Understanding these steps can reassure you about the system’s security protocols and data handling practices.
What future developments do you foresee for neuro-symbolic AI in the legal field?
The future is always brimming with possibilities. What advancements lie on the horizon for neuro-symbolic AI in law? Peeking into future developments can inform you about ongoing trends and potential innovations poised to reshape the legal landscape.
Prescreening questions for Neuro-Symbolic AI for Legal Reasoning
- Can you describe your experience with integrating symbolic reasoning and machine learning techniques?
- What are the key challenges you’ve encountered in developing neuro-symbolic AI systems for legal applications?
- How do you ensure the accuracy and reliability of the AI’s legal reasoning?
- Can you explain how you handle ambiguous or conflicting legal information in your system?
- What methods do you use to extract and represent legal knowledge for neuro-symbolic AI?
- How do you evaluate the performance of your neuro-symbolic AI system in legal reasoning tasks?
- What kind of legal datasets have you used for training and testing your models?
- Can you describe a successful implementation of a neuro-symbolic AI system for a legal domain?
- How does your system handle updates to laws and regulations over time?
- What techniques do you use to integrate case law and statutory law in your reasoning process?
- How do you address explainability and transparency in your neuro-symbolic AI system?
- In what ways does your AI system support decision-making for legal professionals?
- What approaches do you use to ensure compliance with ethical and legal standards in AI development?
- Can your system identify and correct biases within legal data and reasoning processes?
- How does your neuro-symbolic AI system manage the balance between logic-based and data-driven approaches?
- Can you discuss any collaborative projects with legal experts or institutions in developing your AI system?
- How scalable is your neuro-symbolic AI system for different legal jurisdictions and areas of law?
- What are some of the limitations of your current neuro-symbolic AI system for legal reasoning?
- How do you ensure that your system maintains confidentiality and data privacy in handling legal data?
- What future developments do you foresee for neuro-symbolic AI in the legal field?
Interview Neuro-Symbolic AI for Legal Reasoning on Hirevire
Have a list of Neuro-Symbolic AI for Legal Reasoning candidates? Hirevire has got you covered! Schedule interviews with qualified candidates right away.