Prescreening Questions to Ask AI Bias Auditor
Welcome to our comprehensive guide on prescreening questions to ask when you're focusing on identifying and mitigating bias in AI models. Whether you're a hiring manager, a tech enthusiast, or someone keen to understand AI bias, these questions can help you delve deeper into the candidate's expertise and approach. So, let's get started, shall we?
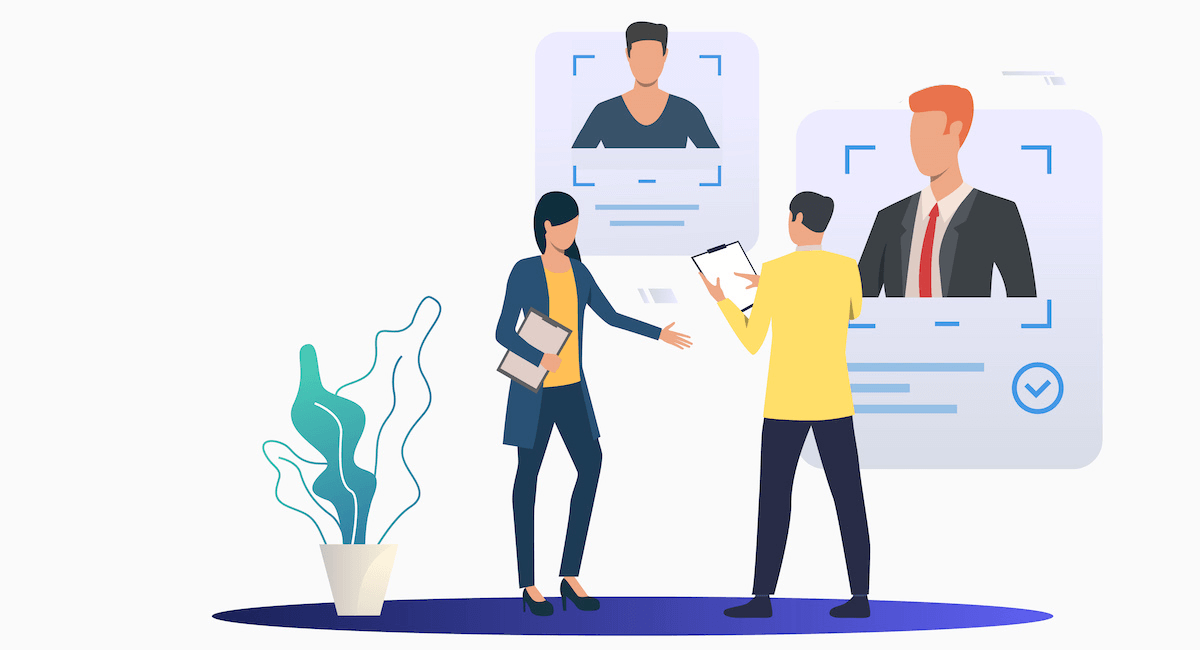
Can you describe your experience with identifying and mitigating bias in AI models?
Getting straight to the heart of the matter, it’s essential to understand someone's background. Have they been in the trenches, slugging it out with biased data? Or are they more of a newcomer? Here, you'd want to hear about specific projects they’ve tackled, the kind of biases they found, and the strategies they employed to fix them.
What methodologies do you employ to detect bias in machine learning algorithms?
There’s more than one way to skin a cat, and the same goes for detecting bias. Do they prefer statistical methods, like disparate impact analysis? Or do they lean on more advanced techniques like adversarial debiasing? Their preference can tell you a lot about their depth of knowledge and their practical experience.
How do you define bias in the context of AI systems?
You’d be surprised how differently people interpret “bias.” For some, it’s an imbalance in training data. For others, it’s systemic issues that trickle down into algorithms. Understanding their definition can help you see if they're on the same wavelength as your organization’s values.
Have you previously conducted audits on AI systems? If so, can you provide examples?
Real-world examples provide tangible evidence of expertise. Maybe they've done a deep dive into a financial institution's decision-making algorithms or evaluated healthcare data for demographic disparities. Their past as a detective in the world of AI bias will give you insight into their skills and problem-solving abilities.
What tools or frameworks do you prefer for auditing AI for fairness and bias?
Tools are a vital part of any AI professional’s toolkit. Do they swear by AI Fairness 360 or Google’s What-If Tool? Or perhaps they have a customized suite of scripts and methodologies? Knowing their preferred tools can give you a peek into their workflow and efficiency.
How do you stay updated on the latest research and developments in AI bias and fairness?
AI is a rapidly evolving field, with new breakthroughs and papers coming out almost weekly. Do they follow specific journals, attend conferences, or read influential blogs? Their answer will tell you how committed they are to staying on the cutting edge of AI bias research.
Can you explain a time when you successfully identified and addressed bias in an AI system?
This is the moment where they shine (or stumble). Practical, hands-on stories can be quite telling. Maybe they discovered that an AI model was less accurate for certain age groups, and then they rebalanced the training data. Stories like these can illustrate their problem-solving prowess.
What metrics do you use to evaluate bias in AI models?
Metrics are the bread and butter of bias detection. You could hear about anything from precision and recall to fairness measures like equalized odds or demographic parity. Their preferred metrics can showcase their analytical skills and depth of understanding.
How do you approach the challenge of balancing fairness and model performance?
This is where the rubber meets the road in AI ethics. Balancing fairness and performance is a tricky tightrope. Do they prioritize fairness even if it means sacrificing some accuracy? Or do they aim for a balanced trade-off? Their stance can highlight their ethical priorities.
What are the common sources of bias in AI systems that you've encountered?
Bias can originate from data collection methods, societal biases, or even from the algorithms themselves. Their insights into common bias sources can help you gauge their thoroughness and attention to detail.
How do you handle bias that arises from imbalanced or unrepresentative training data?
Unbalanced data is like trying to create a masterpiece with only half the colors on your palette. How do they rectify such situations? Do they collect more data, employ synthetic data generation, or adjust their models? Their strategy will reveal their technical adaptability.
Can you discuss how bias can impact different demographic groups in AI applications?
AI bias can have real-world consequences, affecting job prospects, loan approvals, and even judicial outcomes. How well do they understand these implications? Their answer can show their awareness of the broader societal impact of their work.
What experience do you have with regulatory and compliance standards related to AI ethics?
Compliance isn’t just a box-ticking exercise. It’s about ensuring that AI models adhere to ethical standards and avoid discriminatory practices. Their experience with regulations, like GDPR or other ethical frameworks, will show how seriously they take these concerns.
How would you explain the importance of AI bias auditing to a non-technical stakeholder?
This is a true test of their communication skills. Can they break down complex concepts into layman’s terms? Explaining the importance of auditing to someone less versed in AI can indicate their ability to advocate for ethical practices effectively across team barriers.
What role do transparency and explainability play in your auditing process?
Transparency and explainability are the cornerstones of trustworthy AI systems. Do they use model interpretability tools like LIME or SHAP? Their approach can reveal their commitment to making AI decisions understandable and accountable.
Have you worked with any specific industries or sectors where AI bias is a significant concern?
Bias isn’t equally critical in all sectors. Whether it’s healthcare, finance, or criminal justice, different industries have unique challenges. Understanding the sectors they’ve worked in can help determine their versatility and relevance to your needs.
How do you approach collaboration with AI developers and data scientists during an audit?
Auditing isn’t a solo job. It requires teamwork and good interpersonal skills. Do they facilitate workshops, use collaborative tools, or employ other strategies to ensure a smooth auditing process? Their collaborative approach can highlight their leadership and team coordination skills.
What steps would you take if you detected bias that stakeholders were resistant to address?
Resistance to change is a common human trait. How do they navigate this tricky terrain? Do they gather more evidence, appeal to ethical guidelines, or find a compromise? Their strategy for overcoming resistance can showcase their problem-solving abilities and ethical convictions.
In what ways do you believe bias in AI can be proactively prevented rather than reactively corrected?
An ounce of prevention is worth a pound of cure, right? From diverse training data to regular audits and continuous education, proactive measures can save a lot of headaches down the line. Their proactive strategies can highlight their forward-thinking mindset.
Can you provide an example of how you communicated audit findings to ensure actionable outcomes?
Findings are only as good as the actions they inspire. Do they write exhaustive reports, create easy-to-understand dashboards, or hold debrief meetings? Their communication practices can underscore their ability to turn insights into real-world improvements.
Prescreening questions for AI Bias Auditor
- Can you describe your experience with identifying and mitigating bias in AI models?
- What methodologies do you employ to detect bias in machine learning algorithms?
- How do you define bias in the context of AI systems?
- Have you previously conducted audits on AI systems? If so, can you provide examples?
- What tools or frameworks do you prefer for auditing AI for fairness and bias?
- How do you stay updated on the latest research and developments in AI bias and fairness?
- Can you explain a time when you successfully identified and addressed bias in an AI system?
- What metrics do you use to evaluate bias in AI models?
- How do you approach the challenge of balancing fairness and model performance?
- What are the common sources of bias in AI systems that you've encountered?
- How do you handle bias that arises from imbalanced or unrepresentative training data?
- Can you discuss how bias can impact different demographic groups in AI applications?
- What experience do you have with regulatory and compliance standards related to AI ethics?
- How would you explain the importance of AI bias auditing to a non-technical stakeholder?
- What role do transparency and explainability play in your auditing process?
- Have you worked with any specific industries or sectors where AI bias is a significant concern?
- How do you approach collaboration with AI developers and data scientists during an audit?
- What steps would you take if you detected bias that stakeholders were resistant to address?
- In what ways do you believe bias in AI can be proactively prevented rather than reactively corrected?
- Can you provide an example of how you communicated audit findings to ensure actionable outcomes?
Interview AI Bias Auditor on Hirevire
Have a list of AI Bias Auditor candidates? Hirevire has got you covered! Schedule interviews with qualified candidates right away.